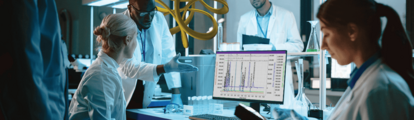
Let’s Make Data FAIR
Embracing accessible, community-supported, interoperable data standards is key to delivering on the promise of Open Science
Frauke Leitner |
sponsored by MilliporeSigma
Collaboration has always been integral to success in science. In the past, collaborators contributed with their knowledge, their instruments, and reports of the results generated. But today, this collaborative spirit also includes the open sharing of the original raw data.
This growing trend towards greater data openness was initially driven by academia, but industry has come to appreciate the immense scientific and commercial potential associated with high quality scientific data accessible to all scientists. Today, there is this wealth of information in databases in the public domain – and it is often the foundation of pharmaceutical R&D.
In this new world of sharing, the pharma industry has typically played the role of data consumer. But that too is beginning to change; many companies now publicly share in-house generated data without the prospect of direct and immediate value – a step forward that was unthinkable not so long ago. However, though companies are increasingly open to sharing data, the community must overcome some technical hurdles – especially, data interoperability – if the Open Science movement is to truly take off. If companies aren’t speaking the same language, willingness to share can only go so far. Instead of using countless proprietary file formats, we need to move towards standards that are accepted across the industry, making both sharing as well as reuse of data easier. And that’s where FAIR comes into play.
Making data FAIR
FAIR stands for findable, accessible, interoperable, and re-usable:
• Findable – discoverable with machine readable metadata, identifiable, and locatable by means of standard identification mechanisms
• Accessible – available and obtainable to both human and machines
• Interoperable – both syntactically parseable and semantically understandable
• Re-usable – sufficiently described and shared with the least restrictive licenses and cumbersome integration with other data sources
Before I go on, allow me to quash the misunderstanding that FAIR data needs to be completely open to everyone and, therefore, it cannot be applied in certain settings, such as in the healthcare industry (where data sets often comprise sensitive personal data) or in the private sector (where data might be subject to intellectual property rights). In fact, FAIR does not require data to be fully open – it simply requires that access conditions for data sets are open and transparent. In practice, data of a highly sensitive nature can be found via its metadata, with access handled very restrictively following evaluation by an ethics committee.
Despite some misunderstandings, we are seeing increasing adoption of FAIR principles as part of the general embrace of Open Data. Over the past five years, the debate has evolved from a question of whether to implement FAIR to how to implement FAIR. The major barrier is a lack of experience – in-house solutions often only address part of the problem and serious discussions are needed to address a couple of questions: Does everything need to be FAIR? What kind of data and metadata provides real added value? FAIRification of data is a journey, and the level of FAIRness that is required depends on the specific use case. Elements that can help to improve FAIRness are identifiers to make data findable, authorization mechanisms to allow accessibility, data standards, and ontologies to make data interoperable.
A different AnIML
The Open Science vision of the future – with companies sharing raw data for the betterment of all – is something we should all be aiming towards. Unfortunately, many companies don’t even do a very good job of sharing data within their own organization – and this lack of data interoperability can have significant impacts on scientists carrying out their daily work. In many organizations, individual experimental results are shared with colleagues via written reports summarizing the main findings. Usually, this is siloed, where colleagues across the floor don’t have access to the original data because it is saved in proprietary files, which only certain people in an organization can open.
The current trend, in line with FAIR principles, is to move towards converting data into open, accessible, and community-supported data standards – an interoperable approach. One such standard is AnIML, the open- source XML standard supported by ASTM International. AnIML provides standardized ways of applying digital signatures to scientific data (which some regulations require), offers the ability to record changes to AnIML documents as part of a built-in audit trail, and includes Experiment Steps that document how a particular analytical technique has been applied to a sample – a basic building block in any analytical workflow.
AnIML is a standard for analytical and biological data, but since AnIML files can – in principle – capture data from any scientific technology, efforts are ongoing to drive adoption of the AnIML standard across a wide variety of scientific domains.
Sharing prosperity
In the public sector, scientists applying for funding are increasingly expected to provide a data management plan along with their application, detailing how they plan to store their data, what metadata needs to be tracked, where the final data set will be published, and which data standards they will implement. This example shows how people can be encouraged to embrace a “data first” mindset, which might be tedious at first but provides valuable guidance and structure, while also supporting good practice in data management – all of which benefits Open Science.
The same forward-thinking mindset can also be applied to the choice of data format – in both the short and long term. In the short term, increasing interoperability and secondary re-use of data is key. In the long-term, you want to be able to open your files in 30 years from now without the need to maintain outdated software for the sole purpose of opening your files locked in proprietary formats (a problem in many organizations!). Converting your data at the point of creation or after initial processing into an open-source XML standard – like AnIML – provides a solution to both your short- and long- term storage needs.
The movement towards FAIR data is picking up speed as almost everyone agrees that we must keep striving towards high-quality, interoperable, open data standards that are supported by the community and across scientific disciplines. But we’re only at the beginning of the journey – companies have a great deal of work to do to define the right level of FAIRness for their data. Regardless of your role within the organization, you can start a discussion on FAIRification of data or participate in already ongoing efforts. Sooner or later, this topic will concern many stakeholders across an enterprise – including lab managers, researchers, data scientists, and QA/QC experts.
For many companies, immediate benefits spring from embracing new ways of collecting and making data accessible to colleagues. And others may have bigger dreams; what questions currently facing humanity will the availability of your data answer in the long-term? In either case, making data FAIR is key.
Product Manager, Connected Lab, MilliporeSigma KGaA, Darmstadt, Germany