Five Digitalization Tips
And why automating workflows in analytical data management is crucial
Richard Lee | | 3 min read | Practical
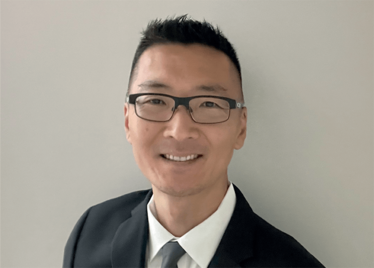
Digitalization of analytical data has been a topic of conversation for many R&D organizations. However, the level of effort exerted – and, therefore, how far each organization is down the digitalization path – varies. For most organizations, the ultimate end goal is to use the data for business intelligence or machine learning frameworks to provide scientific insight beyond what scientists extract from these datasets on a daily basis. But before organizations can start benefiting from digital analytical data, they need to address infrastructure and systems required.
Until recently, organizations thought only of making data available to scientists in the laboratory – to ensure it is assembled in a manner that can be used efficiently to make project decisions. Organizations must now also consider data scientists who will use the same analytical data (or subsets of it) in a very different manner. To satisfy the need for both human readable data for scientists and machine consumable data for data scientists, appropriate systems must be in place. Automation plays a key role. It’s no longer sufficient to automatically shuffle data from one location to another. Data must be normalized, curated, assembled, and contextualized to be fully usable.
For organizations that are still working on their analytical data management strategy, I’ve collated five important considerations.
1. Start simple
Consider the complexity of your workflows. Although it may seem intuitive to tackle complex workflows with automation, they might not necessarily be the best place to start. As complexity increases, the time it takes to document detailed requirements and implement a solution also escalates, slowing your digitalization plan.
Instead, keep it simple by thinking about how you can get “the biggest bang for your buck.” Evaluate the number of data files the workflows of interest generate on a daily basis, as well as the number of manual touch points. These may include data review, data processing, data verification, data transfer, and results entry. Then select to automate workflows that will provide the largest impact – not necessarily the most complex.
2. Consider the needs of your data scientists
For data scientists to leverage analytical data, it needs to be engineered in a format that can be consumed by machines, such as JSON or XML. Automation can help address this critical role of constructing data to a suitable form. Data scientists cannot use the analytical data in a raw or processed format, or even in the “assembled” context of a study (consisting of several analytical data files), they need to use the abstracted results of these studies.
3. Start with the future in mind
Consider how quickly the implemented solution plan can be “cross-pollinated” to similar workflows in adjacent labs or sites – with minimal effort or change. Also remember to build automation services that are scalable so that they are able to address the workflows not only of today, but also as your organization grows with personnel, instruments, labs, and sites. Always plan for the future state to minimize continual iterations.
4. Don’t forget data visualization needs
One aspect that is often overlooked is the user experience. How the data needs to be presented will be dependent on the end user: Scientists will want to view data such as chromatograms and spectra, whereas data scientists will need it formatted differently. Regardless of the type of system or vendor you decide to use, critical scientific decisions will be made by these scientists – as such, it is imperative to implement systems that will benefit both workflows.
5. Start now, and don’t overthink it
There can be a tendency to think and rethink the details, but don’t over-analyze your plans. Don’t be paralyzed by them. Data management will be an iterative process of planning and refinement through configuration, customization, and deployment. You may not have the perfect plan, but it doesn’t need to be.
Issues will arise – integration to other systems, unanticipated system upgrades and more. Accounting for all these variables at the outset will be next to impossible. Don’t over-engineer your plan. More often than not, a well-executed basic plan will start your digitalization journey on the right path.
Richard Lee is the Director of Core Technology and Capabilities at Advanced Chemistry Development, ACD/Labs, Toronto, Canada.