Hypersimple Hyperspectral Imaging
A new deep learning method developed by the University of Illinois allows for low-cost, non-invasive quality assessments of agricultural products using standard RGB photos
| 2 min read | News
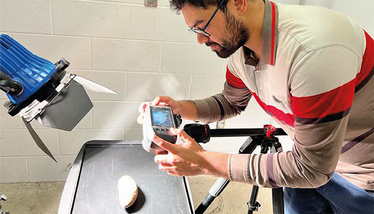
Md Toukir Ahmed, doctoral student at the University of Illinois Urbana-Champaign, takes a photo of a sweet potato with a hyperspectral camera. Credit: College of ACES
A new deep learning method allows researchers to reconstruct hyperspectral images from standard RGB photos, making it possible to conduct low-cost, non-invasive quality assessments for agricultural products. This approach, developed by a team at the University of Illinois Urbana-Champaign, offers potential for simplifying and reducing the costs of product analysis in the agricultural industry.
“Hyperspectral imaging uses expensive equipment. If we can use RGB images captured with a regular camera or smartphone, we can use a low-cost, handheld device to predict product quality,” said lead author Md Toukir Ahmed, a doctoral student in the Department of Agricultural and Biological Engineering (ABE), in a press release.
Hyperspectral imaging (HSI) traditionally requires bulky and costly equipment to capture detailed chemical and physical properties of agricultural products, such as soluble solid content (SSC) and dry matter in crops like sweet potatoes. To overcome these limitations, the research team applied a deep learning model – Hyperspectral Convolutional Neural Network-Dense (HSCNN-D) – to RGB images, enabling the prediction of chemical attributes that typically require hyperspectral imaging.
“With RGB images, you can only detect visible attributes like color, shape, size, and external defects; you can’t detect any chemical parameters,” said Mohammed Kamruzzaman, assistant professor in ABE and corresponding author on the study, in the press release. “But with hyperspectral images, you have many channels and wavelengths from 700 to 1000 nm. With deep learning methods, we can map and reconstruct that range so we now can detect the chemical attributes from RGB images.”
The researchers tested their method on three sweet potato cultivars – Bayou Belle, Murasaki, and Orleans – using RGB images of 141 samples. By reconstructing the hyperspectral data from these images, they were able to predict SSC with over 70 percent accuracy and dry matter content with 88 percent accuracy. These metrics are important for assessing taste, nutritional value, and processing suitability in agricultural products.
The method was tested using a Specim V10E IQ camera to capture hyperspectral images in the visible to near-infrared range (400 to 1000 nm). The integration of machine learning allowed the team to simplify the typically complex and costly hyperspectral imaging process, enabling faster and more accessible agricultural product assessments.
“Our results show great promise for revolutionizing agricultural product quality assessment. By reconstructing detailed chemical information from simple RGB images, we're opening new possibilities for affordable, accessible analysis,” said Kamruzzaman. “While challenges remain in scaling this technology for industrial use, the potential to transform quality control across the agricultural sector makes this a truly exciting endeavor.”
The researchers also applied their deep learning technique in a separate study to predict chick embryo mortality, showing versatility in agricultural applications beyond crop quality. Their findings suggest that deep learning could play a vital role in both animal and plant product assessment, potentially offering a scalable solution for quality control in industries ranging from food processing to hatcheries.