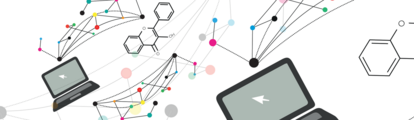
Making Digitalization Work for Everyone
Balancing the needs of experimental and data scientists can pull organizations in different directions. Fortunately, there are digital technologies that suit both camps
Richard Lee | | 4 min read
sponsored by ACD Labs
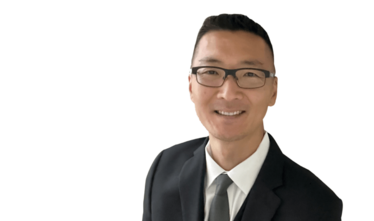
Analytical labs today often have an army of scientific instruments, running 24/7 and generating an immense amount of data. Organizations recognize that there are opportunities to leverage that data to generate additional insights – and there’s a lot of excitement about the possibility of AI and machine learning. But there are also significant challenges.
Lab instruments are often sourced from multiple vendors, each reliant on its own proprietary data format, which creates a lack of interoperability. There are also differences in terms of the data disposition: data generated by one system may be locked into a database, requiring extraction, whereas other systems generate discrete data files. Tackling these issues allows the development of machine learning algorithms, AI frameworks, or other applications for further use downstream. But to get there, data scientists need a representation of the data, abstracted from the individual experiments. They also need remote access, usually via cloud storage.
On the other hand, an organization’s experimental scientists want immediate access to highly interactive data so they can figure out whether their experiment worked and what the next steps are. By moving towards cloud storage, not only are organizations spending a lot of money, but they may be creating some inefficiencies for scientists. Instead of being able to grab data straight from the instrument, they’re having to wait for files to download from the cloud – which may take quite some time for highresolution imaging data, for example.
These two equally valid use cases for analytical data pull organizations in different directions – and finding the right balance can create real headaches. Fortunately, there are technologies available to help organizations make their data work for both camps.
There are now a number of cloud storage providers that have their own object stores – a data storage architecture for storing unstructured data – which organizations can use to store their analytical data. Vendor software can then access that data and pull it back into their own applications via an application programming interface (API). Abstracted data can also reside in data warehouses, which can be leveraged by data scientists for machine learning algorithms.
We’re also seeing the rise of browserbased technologies, which can allow experimental scientists to access their data on demand in a way they’re used to (everyone uses browsers like Google Chrome or Microsoft Edge on a daily basis). ACD/Labs’ Spectrus JS allows scientists to process and interpret their analytical data from any browser, for example.
Automation technology is also making a difference for analytical scientists by stitching together datasets from multiple different techniques and experiments, telling a compelling chemical story. Key here are universal data formats, such as ACD/Labs’ spectrus format. Raw data files can be imported directly to the Spectrus environment and scientists can access their data through the instrument software, process it as they normally would with a single interface, and query by metadata or chemical structures. Processed data files can also be imported into the Spectrus platform, allowing scientists to continue using instrument software they’re used to while being able to assemble and store data from different vendors and techniques together in a scientifically searchable repository. Data scientists can also access the same normalized, contextualized data via an API for machine learning algorithms. Right now, we have a Windows client, but we’re moving towards browser-based access – and that’s very exciting.
Overall, there are technologies available to address the major challenges facing organizations as they embark on their digital transformation journey. I also know that it can be difficult to know where to start, which makes taking those first crucial steps that much harder. My main message? Don’t wait. There are gains to be made today. And there are initiatives to develop standard data formats for all analytical data, which are fantastic (though we’re a long way from ratification). Find a workflow that could see some immediate benefit from digitalization – an area where you know there will be a measurable impact – and start there. You can then expand based on that initial pilot program. I’d also suggest investing in data management and storage, making sure it’s all in one place, properly tagged, and accessible to everyone. This approach puts you on the first step on the ladder so that you’re ready to benefit from AI in the future.
The utopian potential of digitalization is exciting, but it can also be paralyzing. So start small and simple, embrace enabling technology, and set yourself up for success – now and in the future.
Richard Lee is the Director of Core Technology and Capabilities at Advanced Chemistry Development, ACD/Labs, Toronto, Canada.