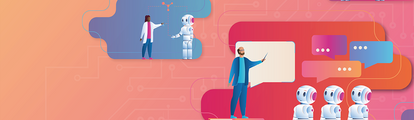
Talking AI with a Big Tech Veteran
Why machine learning expert and former Metaverse and Apple AirPod engineer, Lalin Theverapperuma, thinks artificial intelligence-enabled automation will transform life in the analytical lab – allowing analytical scientists to eliminate mundane tasks and focus on more creative work
James Strachan | | 9 min read | Interview
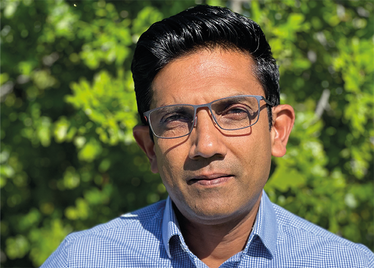
Image Credit: Supplied by Interviewee
Meet the Expert
I’m an engineer through and through. I got my PhD from the University of Minnesota working on machine learning and adaptive signal processing. For the past two decades, I worked at Robert Bosch for about four years, then Intel, and then Apple, where I led the team on the audio and signal processing for the AirPods project – I think people would be surprised if they knew how much signal processing and deep machine learning goes into these products! Then I created a startup company in the automated robotics space, and, after that, I joined Meta to work on hybrid machine learning for Metaverse. More recently, I decided to leave Big Tech to co-found a startup company called Expert Intelligence, where I work as CTO.
Tell me about Expert Intelligence…
Having worked at Apple, Meta, and other leading tech companies, I learned how to build sophisticated and cutting edge AI Tooling. At Expert Intelligence, we have distilled the process ‘how’ to build AI Tooling in order to enable anyone without coding or AI background, to create their own AI-based solution to whatever problem they might need to solve. For example, in chromatography, bioanalytical scientists have built expertise required to accurately integrate peaks - through Expert Intelligence, they can now build custom AI models trained on their data only to automate result interpretation and accelerate the decision making process with higher confidence. This capability is made possible by enabling direct interaction between the analytical expert and the AI module, providing complete transparency and giving the expert full authority to review AI learning events.
We’re speaking at Pittcon… How did you find yourself in the analytical science world?
Actually, this was something of an accident! During the COVID-19 years, we had a client – a group of analytical scientists without a programming background – who asked if we could create AI system for them. So we did that and they were really happy with what we came up with, and we wondered whether there might be more unmet needs in analytical science. It turns out that many people in analytical science spend quite a bit of time doing mundane work that we felt AI could help to automate. After successfully working with more clients in this area, we realized we were onto something, so we decided to focus on this market – hence why I’m here at Pittcon!
I’m new to this area, but having worked on signal processing, the way analytical scientists will look at chromatograms and compare signal to noise ratios immediately resonated with me.
How did you go about creating your EI Platform?
First of all, we wanted individuals without a programming background to be able to engage with our platform, so we focused on the graphical user interface. That interface had to allow the scientist to tell the AI what is important – what noise looks like, what’s critical, and so on. The AI must be able to capture the knowledge the expert is inputting to create a model very quickly, without the need for labeling or annotation – you don’t want to be doing that yourself; trust me!
To do this, we use generative AI. Similar to how DALL.E creates images based on text prompts, our generative AI module learns from small datasets. So it’s a combination of machine learning and signal processing to solve the specific problem. We embed this generative model into an expert-friendly user interface, an automated lab assistant known as EI Co-Pilot. Each EI Co-Pilot is customized to fit the customer's data.
Is it similar to tools like ChatGPT?
A model like ChatGPT is trained with five petabytes of data over weeks – which costs millions. This is a very generic model for general purposes. In the analytical lab, you’re dealing with much smaller and specialized sets of data. In this world, you need precision. For ChatGPT, 90 percent accuracy might be acceptable, but for an analytical science AI tool, you want 99-plus percent accuracy. So that’s a big differentiator – and a challenge. Our idea was to create an AI architecture that allows analytical scientists to develop AI-powered methods tailored to their specific data environment. For example, we’ve helped customers make functional models with around 30–50 samples-worth of raw data; of course, if you have 1,000 samples, the accuracy will be much higher, but there’s a lot AI can do even with smaller data sizes.
There’s a lot of hype around AI right now… But what big analytical problems could AI solve?
I agree that there’s a lot of hype – and concern – around AI. I believe AI can elevate analytical scientists to make data-driven decisions more accurate and confident while automating regular tasks, especially the more monotonous and less creative steps. For example, analytical scientists spend a lot of time looking at analytical data trying to understand spectra; AI can help scientists screen through hundreds or thousands of easily identifiable compounds and find the one that is genuinely tricky. They’ll have to think those through, of course, but much of the routine work could be automated with AI.
The idea is to allow a scientist to be a scientist, as opposed to a data analysis machine. I’ve spoken to some analysts that are looking at 1,800 chromatograms a day. They use the mouse wheel to scroll through because they don’t have time to click. AI can help eliminate this kind of mundane work and the monotonous aspects of people’s jobs, allowing them to focus on the truly meaningful – and difficult – work.
I sometimes say to people, if you can explain your job, or one element of your job, to me, a non expert in their respective field, then AI should be able to help – whether that’s instrument calibration, data processing, data interpretation, or report generation.
What are the main barriers to wider adoption of AI?
Broadly speaking, I think the biggest barrier is how AI is communicated. There are some people who overestimate the potential of AI to tackle problems to which it isn’t well suited – especially given the current hype around tools, such as ChatGPT. But at the same time, you have others who are worried that they’ll be replaced by AI.
We had the exact same conversations around computers when they first came in. Computers have allowed us to automate many tasks and have made our work lives easier in so many ways. Did computers take people’s jobs or allow them to become more productive? It’ll be similar with AI.
Speaking of hype, are there some fundamental limits on what AI can do?
The modern generative AI tools are immediately impressive and, given how early we are in their development, people have very high expectations regarding their future potential. But there are some things that AI struggles with – and will continue to struggle with for the foreseeable future. For example, causality. We all understand that just because the sun comes up when the rooster crows, it doesn’t necessarily mean the rooster is the causal factor. Science-minded people might think the general public isn’t particularly good at understanding the difference between correlation and causation, but we humans are actually very good at it – it is the foundation of our reasoning and our ability to choose one action over another. AI really struggles with causation.
Another connected area is sequential decision making. We don’t appreciate the range of potential options and the whole gamut of possibilities we must take into consideration before making any decision. Outside of a very constrained space like chess or a game of Go, AI struggles to compete with even a toddler when it comes to sequential decision making.
It can be difficult for people to intuit this because it comes so naturally. But when you’re conversing with someone, you both sequentially go from one state to another and then to another, making sequential decisions based on a whole range of factors and possible outcomes. The same thing happens when an analytical scientist is developing a method. For an AI, this is very difficult, it won’t be able to recreate a human’s thought process and reason in this way – at least not any time soon!
What major lessons did you learn in your time in tech?
One big lesson is the importance of taking the time to ensure you find and hire the right people for the job. Though it might sound obvious, I think Big Tech understands this better than most industries. As Steve Jobs said: You have to hire the best. Unfortunately, he didn’t say how! We all know resumes aren’t particularly reliable, so you have to do interviews – lots of them. When I was at Meta, I did 82 interviews to hire just two people. That meant 100s of hours spent interviewing, more time writing up reports for each interviewee, aggravation from management asking why I’m spending so much time on the hiring process... But when you hire someone who immediately becomes a rock star or you build a team that works perfectly together, it can be genuinely transformative for your business or institution. I saw that at Apple as well. They’ve always had a skills and expertise-driven culture with talented people working well together as a team.
Final thoughts on the future of AI – for analytical science and beyond?
I truly believe greater lab automation, facilitated by AI, could be game-changing for analytical science. If there’s one thing I’ve learned, it’s that analytical scientists are doing truly incredible work that leads to the discovery of new medicines, new ways to monitor our health and environment, and so on – but there’s a perception that they’re just in the lab running samples and doing routine work. If we could eliminate the routine work they are doing, it will allow scientists to spend more of their time innovating and creating, which I think will lead to new discoveries.
More broadly, I think the future of AI will be very bright. Overhyped or not, the fact is that many people are putting a lot of money and effort into AI; and history shows us that when this happens, advances are inevitable. We’re still in the very early stages, but I believe we’ll see unmet needs emerging in areas where AI hasn’t yet made a big impact: biology, chemistry, physics, material sciences, and so on. It is an exciting time!
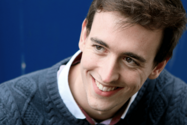
Over the course of my Biomedical Sciences degree it dawned on me that my goal of becoming a scientist didn’t quite mesh with my lack of affinity for lab work. Thinking on my decision to pursue biology rather than English at age 15 – despite an aptitude for the latter – I realized that science writing was a way to combine what I loved with what I was good at.
From there I set out to gather as much freelancing experience as I could, spending 2 years developing scientific content for International Innovation, before completing an MSc in Science Communication. After gaining invaluable experience in supporting the communications efforts of CERN and IN-PART, I joined Texere – where I am focused on producing consistently engaging, cutting-edge and innovative content for our specialist audiences around the world.