The XCMS-METLIN Story
Gary Siuzdak’s team at Scripps Research did more than just process LC/MS data – they mastered the art of distinguishing signal from noise, uncovering molecular identities hidden in the clutter of raw data
Gary Siuzdak | | 7 min read | Review
The origins of The Analytical Scientist’s 2023 Innovation Award-winning XCMS-METLIN platform trace back over three decades. The story begins in the early '90s with a bold idea from Richard Lerner, then president of Scripps. Richard wanted to explore the cerebrospinal fluid of animals in a sleep-deprived state, looking for endogenous metabolites that might induce sleep. My task was to identify these molecules. We initially used GC-MS for the analyses, but this didn’t give us the comprehensive data we needed.
That led to our first LC-MS-based metabolomic and lipidomic experiments, which culminated in several key publications (1,2). We discovered molecules correlating with the sleep-wake cycle, including one that induced a sleep-like state. But those early experiments were plagued by challenges. Data alignment and identification, in particular, posed significant barriers to progress. For example, retention time variability from run to run made it difficult to align data and discern real signals from noise. The complexity of molecular identification was another major hurdle, especially when manual methods were our only option.
The Analytical Scientist Presents:
Enjoying our content? Join a growing community of like-minded individuals with the hottest topics at your fingertips, specially curated by our Editorial team.
The birth of XCMS – and nonlinear retention time alignment
The breakthrough came in the early 2000s when I challenged Colin Smith, a talented staff scientist, to improve our data analysis methods. The result? XCMS (3). XCMS introduced a novel concept: nonlinear alignment of LC/MS data. This allowed us to adjust for variations across experiments and vastly improved our ability to distinguish real signals from noise.
The first XCMS nonlinear correction plot (Figure 1) and algorithm, which addressed the challenge of LC/MS drift, has since become a widely emulated approach across the field. And given the large population of XCMS users, we are constantly listening to their thoughts on how XCMS can be improved. For example, pairwise analysis was always standard, however, the addition of XCMS single sample analysis and XCMS multi-group analysis came from user input.
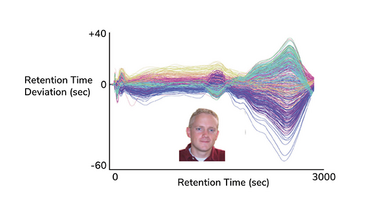
Figure 1. Derived from the first output from the XCMS program, retention time deviation versus retention time (Colin Smith inset), and what turned into the XCMS logo. (Analytical Chemistry 2006)
Enter METLIN – the gold standard for molecular identification
Even with XCMS’s alignment solutions, we still faced the challenge of identifying the myriad peaks from LC/MS data. Accurate mass measurements alone proved unreliable because isomers and isobaric compounds – like glucose, lactose, and fructose – share identical molecular weights. We needed something more.
That "something" was tandem mass spectrometry (MS/MS), which provides an additional level of molecular characterization. This realization led us to create METLIN – a comprehensive MS/MS database.
Initially, METLIN was built by collecting known endogenous metabolite and lipid standards, generating MS/MS data at multiple collision energies (0, 10, 20, and 40 eV). Over the first decade, METLIN cataloged over 10,000 molecules, growing to almost 20,000 in the second decade. Today, METLIN hosts MS/MS data on over 935,000 molecular standards from over 350 classes of molecules (Figure 2), this represents exponential growth made possible by solving three key challenges:
- Acquiring molecular standards: We gathered a vast range of molecules from over 350 chemical classes to populate METLIN, the acquisitions occurred from individual labs, chemical companies, and pharmaceutical firms. (Special thanks to Avanti Polar Lipids for the vast store of lipids they provided (Figure 3).)
- Automating data acquisition and maintaining data quality: High-throughput analysis capabilities emerged after a major lab flood in 2017 destroyed several of our instruments. Ironically, this disaster enabled us to rebuild with even better equipment and higher efficiency, translating into quality data generation during high throughput analyses (Figure 4).
- Informatics integration: Aries Aisporna, a key team member, deserves much credit for creating informatics solutions that allowed us to simultaneously process molecular information, guide the analyses, and integrate everything into a user-friendly platform.
Winnie Uritboonthai also played a critical role, optimizing our mass spectrometry systems and processing over a million molecules with a success rate of around 80 percent. Thanks to her tireless efforts, METLIN has become the gold standard for MS/MS data, with experimental data for over 935,000 molecules at various collision energies.
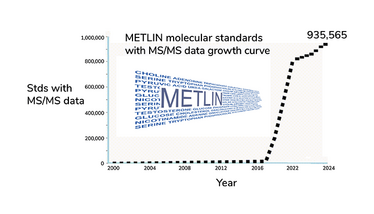
Figure 2. METLIN was created in the early 2000s initially with primary metabolites and lipids, and has since grown to contain over 935,000 molecular standards each with MS/MS data at multiple collisional energies. Therapeutic Drug Monitoring 2005, Nature Methods 2020, Mol. Sys. Biol. 2024
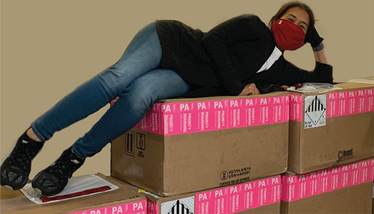
Figure 3. Pandemic era shipment of lipid molecular standards from Avanti Lipids; Winnie Urtiboonthai inspecting the delivery.
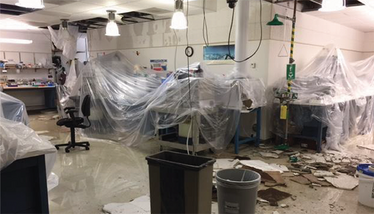
Figure 4. The flood of 2017 caught on video, filled the lab with 10,000 liters of water.
Machine Learning and In-Silico Data: A Cautionary Tale
A few years ago, we explored the potential of machine learning to predict fragmentation patterns and generate in-silico data to supplement METLIN. For a brief period, we even included this predicted data in the database. However, it quickly became clear from the users of METLIN that the technology wasn’t ready. False identifications were rampant, misleading users of METLIN and sending them down the wrong paths. Users ultimately convinced us to remove in silico generated data.
This experience was a stark reminder that even in an era of rapid technological advances, experimental data remains paramount. Today, METLIN contains only experimentally verified data, and I believe this decision has safeguarded the platform's integrity.
XCMS-METLIN’s impact – science and beyond
If I had to distill the real value of XCMS and METLIN, it would come down to their impact on science. These platforms have catalyzed key breakthroughs:
- XCMS: Nonlinear alignment of LC/MS data (3)
- METLIN: Streamlined molecular identification (4,5,6)
- Activity Metabolomics: Enabled by XCMS and METLIN (7,8,9,10)
- Phantom Metabolites Unveiled: An intriguing discovery from METLIN (Figure 5) is that much of the LC/MS data we once treated as meaningful is, in fact, noise – caused by in-source fragmentation (ISF). Through METLIN’s unique acquisition of data at 0 eV, we have been able to distinguish true molecular ions from these fragments, simplifying our understanding of the metabolome and lipidome (11).
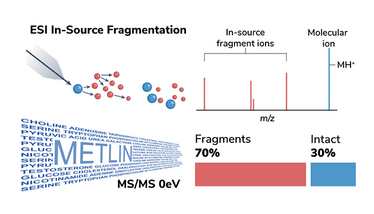
Figure 5. In-source fragmentation occurs when small molecules break apart within the mass spectrometer before mass analysis. This process, known since the early days of electron ionization, is also common with electrospray ionization (ESI). In ESI, fragment ions are generated and detected alongside intact molecules, adding complexity to LC/MS data. These fragments complicate interpretation because they represent portions of molecules rather than the entire entities, making it harder to determine the exact nature and quantities of substances in a sample. In-source fragmentation has long been a source of ambiguity in LC/MS data.
Beyond its scientific contributions, XCMS-METLIN has had significant commercial impact. Early cloud-based versions of XCMS have raised concerns about data privacy, especially for industry users. In response, our local version of the new XCMS-METLIN platform (Figure 6), allows companies and institutes to process their data in-house with the major added advantage of streamlined molecular identification with an unrivaled database.
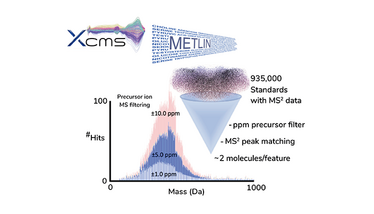
Figure 6. The integration of XCMS and METLIN as a local platform provides a streamlined means of LC/MS analysis and molecular identification, all within the confines of a personal computer.
But if there’s one thing I’ve learned throughout the development of XCMS-METLIN, it’s that no innovation happens in isolation. I’ve simply learned to listen and value the ideas of others – especially from brilliant scientists like Colin, Aries, and Winnie. XCMS-METLIN is the culmination of a tremendous team effort.
Image credits: Supplied by Author
- RA Lerner et al., “Cerebrodiene: a brain lipid isolated from sleep-deprived cats,” Proc Natl Acad Sci USA, 91, 9505 (1994). DOI: 10.1073/pnas.91.20.9505.
- BF Cravatt et al., “Chemical characterization of a family of brain lipids that induce sleep,” Science, 268, 1506 (1995). DOI: 10.1126/science.7770779.
- G Siuzdak, “Mass spectrometry: an evolving tool for metabolomics,” Anal Chem, 78, 413A (2006). DOI: 10.1021/ac051437y.
- G Siuzdak et al., “METLIN: a metabolite mass spectral database,” Ther Drug Monit, 27, 747 (2005). DOI: 10.1097/00007691-200512000-00016.
- J Xue et al., “METLIN MS2 molecular standards database: a broad chemical and biological resource,” Nat Methods, 17, 953 (2020). DOI: 10.1038/s41592-020-0942-5.
- M Giera et al., “XCMS-METLIN: data-driven metabolite, lipid, and chemical analysis,” Mol Syst Biol, 20, 1153 (2024). DOI: 10.1038/s44320-024-00063-4.
- BF Cravatt et al., “Chemical characterization of a family of brain lipids that induce sleep,” Science, 268, 1506 (1995). DOI: 10.1126/science.7770779.
- C Guijas et al., “Metabolomics activity screening for identifying metabolites that modulate phenotype,” Nat Biotechnol, 36, 316 (2018). DOI: 10.1038/nbt.4101.
- C Guijas et al., “Metabolomics activity screening for identifying metabolites that modulate phenotype,” Nat Biotechnol, 36, 316 (2018). DOI: 10.1038/nbt.4101.
- MM Rinschen et al., “The functional role of metabolomics in systems biology,” Nat Rev Mol Cell Biol, 20, 353 (2019). DOI: 10.1038/s41580-019-0108-4.
- M Giera et al., “The hidden impact of in-source fragmentation in metabolic and chemical mass spectrometry data interpretation,” Nat Metab, 6, 1647 (2024). DOI: 10.1038/s42255-024-01076-x.
Gary Siuzdak is Professor and Director of the Scripps Center for Metabolomics at Scripps Research, La Jolla, California, USA.