FAIR Game
Why Open and FAIR data sharing in analytical research is important for public data availability, raising awareness of your data, and the very future of analytical science
Emma L. Schymanski | | 5 min read | Opinion
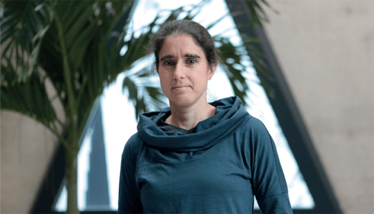
Open and FAIR data seem all the rage right now – but what lies behind the jargon and the hype about FAIRification and FAIRifying data? What is the difference between Open and FAIR? Do we need one or the other – or both?
The FAIR data principles were published in 2016 and are described on the GO FAIR website (1). Briefly, FAIR means Findable (you can find the data easily), Accessible (you have full access to the data), Interoperable (you are able to use the data in different workflows, operating or storage systems) and Reusable (not only can you find and access it, but you are also able to use it and have sufficient metadata to do so).
Open Data, on the other hand, describes data that is available under an open license and is thus accessible to anybody. The FAIR principles do not necessarily imply “open” – leading to the phrase “as open as possible, as closed as necessary,” which can help when dealing with personal data that is not suitable for public distribution. In this case, it can still be FAIR but “Accessible” behind a login for authorized users, for example.
Open data that is available to everybody, but is not findable, interoperable, or reusable is only of very limited use. Thus, making data both Open and FAIR is more powerful than one or other alone – and although it often requires concerted effort, it offers both collective and individual rewards. The good news is that the infrastructure and training programs to support researchers in sharing FAIR and Open data are getting better by the day!
So, why share data? There are many possible motivations and reasons, ranging from a strong conviction that publicly funded research should be publicly available, or frustration when research ends up behind a paywall, through to getting a buzz out of seeing your own data “come to life” in public resources that are used by millions of people around the world. Making data available under a creative commons license with attribution (stipulating that the authors should be credited) can raise the awareness of your data to other researchers even before publication, and ensures that the contributing authors retain credit for their work. Publishing data in a repository with a clear license and a Digital Object Identifier (DOI) means that this dataset can be citable as an individual entity in the resulting publication as well – helping to track downstream use and interest.
An example of FAIR and Open data is the NORMAN Suspect List Exchange (NORMAN-SLE) – a central access point for researchers to find suspect lists relevant for their environmental monitoring questions (2). One of these lists – list S61 UJICCSLIB (3) – comprises a collision cross section (CCS) values dataset for ion mobility mass spectrometry and was available in PubChem and had over 1,000 downloads before the study was even published (4). The public interest in this dataset helped encourage others to contribute their datasets.
PubChem now contains CCS values for over 5,600 chemicals – with more in the pipeline! This publicly available data is both human and machine-readable and can be extracted automatically and used by researchers around the world to help develop better prediction methods for CCS values. The FAIR metadata within PubChem can also be used to create highly relevant subsets of PubChem to make analytical workflows far more efficient (5). The integration of the NORMAN-SLE into PubChem has resulted in the addition of over 6,000 chemical structures to PubChem that were not otherwise present – including the integration of highly-specialized analytical data (NMR, MS/MS and CCS) from transformation products to aid researchers in identification of these newly discovered substances in their samples.
Has making data Open and FAIR helped me in my own research? Of course – but beyond that, it has also enabled us, as a community, to develop and contribute to the developments of resources that have helped many others as well. Our resources such as MassBank.EU, MetFrag (6), NORMAN-SLE, PubChemLite for Exposomics (7) are built on Open and FAIR data and are likewise Open and FAIR.
By having the privilege of working with so many researchers to help share their data, we have been able to develop some simple guidelines and templates (for chemical structures, transformations and CCS values so far) to assist researchers in providing their data in a manner that allows for better integration into downstream resources (8,9). These templates have been designed to be as FAIR as possible, while keeping things as simple as possible for researchers so that the overall effort required does not become a major barrier to contribution. Most tips are simple, such as using consistent and clear column headers, interoperable formats and identifiers/information that can be readily interpreted in a clear and automated manner, for both humans and machines.
Personally, I am excited to see how Open and FAIR data will transform analytical chemistry in the coming years. In just under a decade, we have witnessed our small community effort grow from being a vague idea to an environmental knowledge base on over 100,000 chemicals (2). If you would like to contribute your data, please consider using the templates mentioned above in your efforts – and if you have other data not covered by these templates, please get in touch, as we are drafting more templates to support researchers in providing analytical data in an Open and FAIR manner!
I hope I’ve helped clarify what Open and FAIR is and, even better, inspired you to consider publishing your data in an Open and FAIR manner. If that is the case, we look forward to seeing your data in the open space soon and hope you will enjoy seeing your research come to life in ways you may have never imagined!
- GO FAIR, “FAIR Principles” (2021). Available at: https://bit.ly/2Qt21U0
- H Mohammed Taha et al., "The NORMAN Suspect List Exchange (NORMAN-SLE): facilitating European and worldwide collaboration on suspect screening in high resolution mass spectrometry," Environmental Sciences Europe, 34, 1 (2022). DOI: 10.1186/s12302-022-00680-6
- A Celma et al., “S61 | UJICCSLIB | Collision Cross Section (CCS) Library from UJI,” Zenodo (2019). DOI: 10.5281/zenodo.3549476
- A Celma et al., “Improving Target and Suspect Screening High-Resolution Mass Spectrometry Workflows in Environmental Analysis by Ion Mobility Separation,” Environmental Science & Technology, 54, 15120 (2020). DOI: 10.1021/acs.est.0c05713
- S Kim et al., “PubChem in 2021: new data content and improved web interfaces,” Nucleic Acids Research,” 49, 1, DOI: 10.1093/nar/gkaa971.
- C Ruttkies et al., “MetFrag relaunched: incorporating strategies beyond in silico fragmentation,” Journal of Cheminformatics, 8,3, (2016). DOI: 10.1186/s13321-016-0115-9
- EL Schymanski et al., “Empowering large chemical knowledge bases for exposomics: PubChemLite meets MetFrag,” Journal of cheminformatics 13, 1 (2021). DOI: 10.1186/s13321-021-00489-0
- EL Schymanski & EE Bolton, “FAIR chemical structures in the Journal of Cheminformatics,” Journal of cheminformatics, 13, 1 (2021). DOI: 0.1186/s13321-021-00520-4
- EL Schymanski & EE Bolton, “FAIR-ifying the Exposome Journal: Templates for Chemical Structures and Transformations,” Exposome 2, 1 (2022).DOI: 10.1093/exposome/osab006
Associate Professor, Luxembourg Centre for Systems Biomedicine (LCSB), University of Luxembourg, Belvaux, Luxembourg