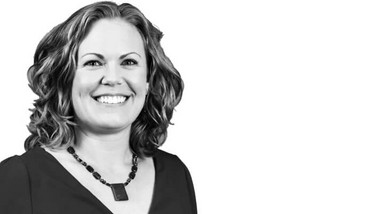
The institutions funding our research and journals publishing our discoveries make, almost without exception, a seemingly simple request: make your science reproducible. Why? Because we owe it to the taxpayers – who often foot the bill – to make the best possible use of their money, and we owe it to our colleagues to ensure that they can trust our conclusions.
To ensure reproducibility, we perform replicate experiments, report standard deviations and conduct significance testing. We also name the sources of chemical standards, reagents and cell lines, document the instrumentation used and software deployed, detail method parameters and cite the studies central to method development.
This information alone, however, is not enough for one to reproduce a given experiment. Consider the following: you ask your most senior researcher to reproduce the results of a paper they’re submitting for publication, relying only on the methods section (that is, they can’t use the saved method on the instrument software). The result? Disaster! In reality, nobody reports all experimental details, and there are several reasons for this.
Journal articles are restricted regarding their length and formatting. Utilizing supplementary information to provide a more complete overview of methodology has become the workaround of choice; yet, typically this amounts to nothing more than endless lists of parameters in narrative format – hardly easy to interpret, particularly for the non-expert. The ever-growing availability and adoption of black-box methods with hidden default settings that can be difficult to deduce and report is then another issue. Consider a parameter as ubiquitous as a signal-to-noise threshold in a data processing method, which can be calculated in multiple ways, but is rarely described in the software manual.
The rise of interdisciplinary science has introduced more diverse and complex methodologies. The methods outlined in manuscripts for biomarker discovery and validation studies, for example, would include (and may not be limited to): study design, subject recruitment, subject and sample characteristics, sample handling and processing, chromatography, MS, data processing, data post-processing, statistical methods, machine learning, and validation steps. The burden of ensuring that every one of these steps is thoroughly reported ultimately falls on the journal’s reviewers, but – realistically – it’s highly unlikely that the review team will cover all of the necessary expertise.
Bench scientists and reviewers alike suffer from poorly reported methods; I myself have had difficulty in citing a standard data processing parameter due to a lack of previous reports, leaving both myself and the requesting reviewer at a loss. Such issues highlight a central question that we must answer moving forwards: how do we ensure that we, as a community, make our experiments truly reproducible?
As authors, tables and lists are our friends. Not every methodological parameter will fit this style, but many will, and for investigators trying to adapt them to their own software platforms, it is much easier to match against these formats. For greater transparency, why not print the methods, scripts and/or code directly out of the software and provide those printouts as supplementary files? This also reduces the probability of errors.
The responsibility is not, however, that of the authors alone. Reviewers must critically appraise the methods sections of submissions and request more detail where they deem an experiment cannot be reproduced from the manuscript content. To this end, publishers themselves must be more specific in their standards for methods sections and should hire staff with the necessary experience to ensure these standards are fit for purpose in our ever-evolving field.
Software manufacturers can also play their part by streamlining the export of method tables in editable and easily interpreted formats. These tables should also include the default parameters not editable by users. The software should track the provenance of raw, processed and post-processed data and generate reports on how data has been integrated, transformed and filtered.
If we want to provide a firm foundation for the researchers of tomorrow to build on, we need to accept that reproducibility is everyone’s responsibility.
Assistant Professor, School of Life Sciences, Arizona State University, Arizona, USA.