AI-Enabled Precision Drying
New system combines spectroscopic imaging and machine learning to monitor food drying in real time
| 2 min read | News
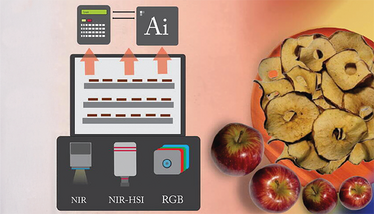
A new food drying system integrates hyperspectral and fluorescence imaging with machine learning to enhance the precision and efficiency of drying processes. This non-destructive approach offers real-time insights into key parameters such as moisture content and drying rates, addressing challenges in maintaining food quality and nutritional value during processing.
The system uses hyperspectral imaging to collect detailed spectral data across a wide range of wavelengths, detecting subtle chemical and physical changes during drying. Fluorescence imaging identifies biomarkers linked to product quality. Machine learning models analyze the extensive datasets produced by these methods, enabling accurate and continuous monitoring.
“With traditional drying systems, you need to remove samples to monitor the process. But with smart drying, or precision drying, you can continuously monitor the process in real time, enhancing accuracy and efficiency,” said corresponding author Mohammed Kamruzzaman, assistant professor in the Department of Agricultural and Biological Engineering (ABE) at the University of Illinois Urbana-Champaign, USA, in a press release.
During experiments, the researchers applied their system to dry apple slices, a common benchmark for testing food drying techniques. RGB imaging captured surface-level features such as size, shape, and color, while near-infrared (NIR) spectroscopy measured internal qualities like moisture content. Near-infrared hyperspectral imaging (NIR-HSI) provided even greater detail, scanning entire surfaces to combine spatial and spectral data for enhanced precision.
“NIR-HSI is the most comprehensive of the three techniques. It scans the whole surface of the product, so it provides much more precise information about the drying rate and other features than NIR alone,” explained lean author Marcus Vinicius da Silva Ferreira, a postdoctoral fellow in ABE.
The researchers emphasized that each imaging technique has strengths and limitations. While NIR-HSI provides the most detailed data, its high cost – up to 20 times more than NIR sensors – makes it less accessible for all applications. Machine learning algorithms trained on benchmark datasets are required to interpret the data from these sensors, making the system adaptable to various drying setups and food products.
“For real-time monitoring, the convergence of RGB imaging, NIR spectroscopic sensors, and NIR-HSI with AI represents a transformative future for food drying,” the team concluded in the paper.
Going forward, the researchers are aiming to create portable, handheld NIR-HSI devices to enable on-site quality monitoring, providing real-time control for drying systems in diverse industrial environments.