Making Sense of Metabolite Mixtures
Substructure analysis provides a means to decipher complex metabolic samples.
Justin J.J van der Hooft | | Opinion
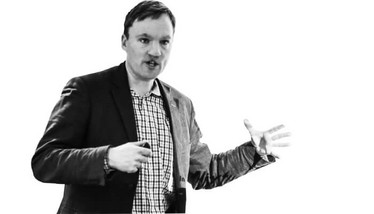
credit: Elodie Burrillon
Personalized medicine. Antibiotic discovery. Host–microbiome interactions. Plant breeding. What do these fields have in common? All have benefited from the realization that comprehensive small molecule profiles are essential to increase our knowledge of complex biological systems. Metabolites then represent key markers in such studies, and are often the end goal of analyses.
To study metabolites, scientists typically extract small molecules from biological systems and subject them to MS. In recent years, technological advances have supercharged this technique; we are now able to analyze huge numbers of different molecules and vital data for their structural elucidation, such as their so-called fragmentation spectra, are more easily obtained. Faster measurements mean more spectral data, and ongoing optimization in this space means we are able to collect more than ever before. Yet, despite our ability to obtain a wealth of high-quality spectral data from biological systems, a major challenge remains: translating this spectral data into structural information.
Metabolite annotation and identification represent major bottlenecks when performing such translations (1). The main challenges are the structural diversity of metabolites found in natural extracts and the (still) large number of completely novel molecules measured. For the latter, there are no reference data available – not even from structurally-related molecules. Therefore, it’s not surprising to see such a focus on the linking of spectral data to structural information at both the Faraday Discussions on challenges in analysis of complex natural mixtures (Edinburgh, UK, May 13-15, 2019) and the Metabolomics2019 meeting (The Hague, NL, June 24-27, 2019). Traditionally, structural information is obtained by matching experimental mass spectra with database spectra for reference molecules (2); however, in practice, only a few percent of spectra can then be structurally annotated.
It is my view that metabolome mining and annotation tools will prove to be a crucial toolkit for overcoming this data deluge. How? By translating spectral data into structural information and providing comprehensive yet detailed chemical overviews of natural extracts. These approaches are based on two principles: i) the usual presence of structurally-related molecules in natural extracts, and ii) the similar mass fragmentation spectra of molecules sharing similar structures. In silico approaches hope to extract chemical information from spectral data by finding and recording relationships between spectra, and matching candidate structures with shared building blocks from databases with these measured mass spectra.
Once spectral relationships are known, one can build networks in which structurally-related molecules are grouped into molecular families (3). My team and others have shown that comprehensive chemical pictures and unprecedented chemical details can be obtained from spectral data when applying metabolome mining and annotation tools to complex metabolite mixtures in such a way (4,5,6,7). Moreover, the outcomes of different analysis workflows can be integrated; for example, fragmentation spectra can also be mined for co-occurring mass peaks.
Inspired by text-mining algorithms, the MS2LDA tool was designed to locate the chemical imprints of molecular substructures in metabolomics data using topic modeling (8,9,10). Analogous to searching for trending topics in text documents based on co-occurring words, MS2LDA groups consist of molecules with co-occurring mass peaks that can be linked to relevant biochemical substructures. The MolNetEnhancer workflow (11), which includes the MS2LDA tool, allows researchers to integrate several mining and annotation tools to detect the presence or absence of target substructures within molecular families.
Substructure-based workflows enhance our understanding of chemical profiles – and the profiling process itself – in manifold ways, from grouping unknown chemicals and explaining chemical differences between samples to structural elucidation (12). Extracting chemical information from metabolomics profiles by such methods will then work to bridge metabolomics to the broader omics field in many areas – for example, in matching natural products to the biosynthetic gene clusters behind their production.
All in all, I believe that substructure analysis will play a key role in helping to decipher complex biological systems and the functional role that metabolites play within them. And I look forward to the bright future of this field.
- WB Dunn et al., “Mass appeal: metabolite identification in mass spectrometry-focused untargeted metabolomics”, Metabolomics, 9, 44 (2013). DOI: 10.1007/s11306-012-0434-4
- T Kind et al., “Identification of small molecules using accurate mass MS/MS search”, Mass Spec Rev, 37, 513 (2018). DOI: 10.1002/mas.21535
- M Wang et al., “Sharing and community curation of mass spectrometry data with Global Natural Product Social Molecular Networking”, Nat Biotech, 34, 828 (2016). DOI: 10.1038/nbt.3597
- M Ernst et al., “Assessing specialized metabolite diversity in the cosmopolitan plant genus Euphorbia L.”, Front Plant Sci, 10 (2019). DOI: 10.3389/fpls.2019.00846
- KB Kang et al., “Comprehensive mass spectrometry-guided phenotyping of plant specialized metabolites revelas metabolic diversity in the cosmopolitan plant family Rhamnaceae”, Plant J, 98, 1134 (2019). DOI: 10.1111/tpj.14292
- JL Wolfender et al., "Accelerating Metabolite Identification in Natural Product Research: Toward an Ideal Combination of Liquid Chromatography-High-Resolution Tandem Mass Spectrometry and NMR Profiling, in Silico Databases, and Chemometrics", Anal Chem, 91, 704 (2018). DOI: 10.1021/acs.analchem.8b05112
- AE Fox Ramos et al., “Natural products targeting strategies involving molecular networking: different manners, one goal”, Nat Prof Rep, 36, 960 (2019). DOI: 10.1039/c9np00006b
- JJJ van der Hooft et al., “Topic modeling for untargeted substructure exploration in mdtabolomics” PNAS, 113, 13738 (2016). DOI: 10.1073/pnas.1608041113
- S Rogers et al., “Deciphering complex metabolite mixtures by unsupervised and supervised substructure discover and semi-automated annotation from MS/MS spectra”, Faraday Discuss (2019). DOI: 10.1039/C8FD00235E
- J Wandy et al., “Ms2lda.org: web-based topic modelling for substructure discovery in mass spectrometry”, Bioinformatics, 34, 317 (2018). DOI: 10.1093/bioinformatics/btx582
- M Ernst et al., “MolNetEnhancer: Enhanced Molecular Networks by Integrating Metabolome Mining and Annotation Tools”, Metabolites, 9, 144 (2019). DOI: 10.3390/metabo9070144
- JJJ van der Hooft, “Unsupervised Discovery and Comparison of Structural Families Across Multiple Samples in Untargeted Metabolomics”, Anal Chem, 89, 7569 (2017). DOI: 10.1021/acs.analchem.7b01391
Bioinformatics Group, Wageningen University, Wageningen, the Netherlands.