AI to the Rescue: Tackling the Proteomic Data Deluge
As the depth and throughput of mass spectrometry proteomics increases, so does the complexity and volume of data produced; AI and ML will be key to managing this challenge, aiding in the identification and quantification of proteins at scale and opening up entirely new avenues of research
Lukas Reiter | | 4 min read | Opinion
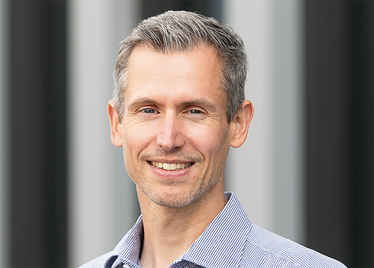
At the turn of the 21st century, the field of mass spectrometry (MS) proteomics was dogged by problems. While RNA sequencing methods could profile thousands of transcripts in human cells more than a decade ago, researchers could still only see the very tip of the proteome iceberg. MS proteomics methods were perceived as having a lack of depth, poor reproducibility, and low throughput, limiting their use in biopharma research.
Now, proteomics is catching up and even starting to surpass other -omics technologies when it comes to revealing the underlying biology of health and disease. Recent technological advancements, such as ultra-deep mass spectrometry, have achieved nearly 100 percent proteome coverage in both cell lines and tissues. Previously undetected low-abundance proteins – the proteins most relevant to disease biology – can now be identified and quantified. But our pursuit of ever deeper coverage has historically come at the expense of throughput.
As a result, the focus of the field has recently shifted towards enhancing throughput, with a number of key advances in this area over the past few years that have made large-scale, proteome-wide analysis a reality.
As we bridge the gap between deep proteome coverage and high throughput – along with lower costs – we will see novel applications opening up for MS proteomics, resulting in more and more data. The solution to this ever-increasing mountain of information? Better data analysis algorithms.
AI algorithms have been a key driver in the deep and efficient analysis of the enormous amount of data generated by modern MS proteomics. Compared with conventional algorithms, AI computing approaches, such as neural networks, can process large amounts of information in parallel, making them highly efficient tools for data analysis.
But the sheer volume of data isn’t the only issue – the information generated by modern mass spectrometry is also incredibly complex. This information can be pictured as a multitude of peptide data points spread out in multi-dimensional space, and precise coordinates are needed to efficiently identify them. With this level of complexity, trying to maintain accuracy and reproducibility is challenging because the data is always changing – in response to novel instrumentation, for example.
Thankfully, AI algorithms are highly adaptable, extracting the maximum amount of valuable information from the data. As I covered in my talk at last year’s Human Proteome Organization (HUPO) World Congress, one way to achieve this adaptability is through an approach known as transfer learning.
Transfer learning enlists the help of pre-trained neural networks to refine and improve predictions about the protein composition of a sample based on the data currently being analyzed. In practice, this means analytes identified with high confidence in a first pass can be used for transfer learning, maximizing the output of a final analysis. And with modern tools, this process can be automated, eliminating the need for pre-existing libraries.
As AI and machine learning approaches continue to improve, it is likely we will be able to identify more and more analytes from a given LC-MS acquisition. It is also likely that throughput will continue to double every two years – a trend we’ve seen over the past decade. In these conditions, quantification becomes increasingly important.
AI and deep learning tools can greatly improve the accuracy of quantification by deconvoluting overlapping or interfering signals within MS data. This is significant as interference is particularly problematic for low abundance proteins, where the majority of biologically relevant biomarkers tend to be. For example, our own in-house neural network, DeepQuant, applies deep learning to correct for interferences, picking out the signals from the noise to improve the quantification of low abundance proteins.
Vastly improving the quantification of proteins through the use of AI and ML tools could mark the single biggest step change we see in MS-based proteomics over the coming years. We’ve already seen significant progress over the years in terms of throughput, depth, and cost – now researchers have the tools to navigate the complexities of data analysis and unlock previously unattainable insights.
Entirely new proteomics applications are now conceivable, such as cell line screens or large-scale mechanism of action studies, and we could even see an impact on clinical diagnostics or the approval of drugs based on biologically meaningful surrogate biomarkers further down the road. In this way, AI and ML tools are set to completely reshape the perception of what MS-based proteomics is and what it can achieve.
Image Credit: Supplied by Author
Chief Technology Officer at Biognosys