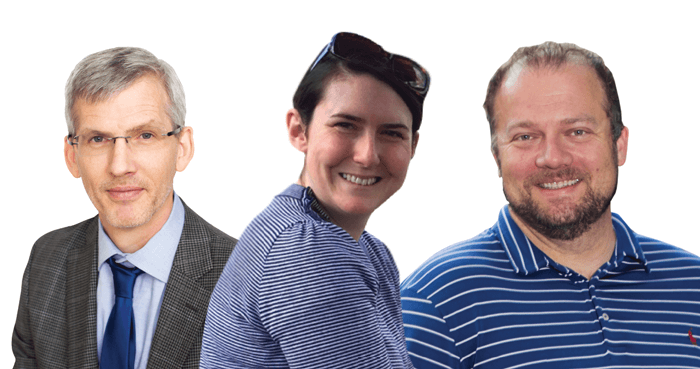
What is the single biggest challenge in analytical data management?
Graham: The challenge of data heterogeneity is real (1) – and it was highlighted in the survey from ACD/Labs and The Analytical Scientist’s on analytical data management (see the infographic below for the headline figures). Labs are often dealing with a variety of different vendor technologies, software, and hardware all collecting data, which makes summarizing the information difficult. And that’s just for a single technique – often people are using two or more techniques for a given analytical question.
Mark: Yes, I can relate to that! At our global Research and Innovation centers with Solvay, not only do we have a whole plethora of techniques to contend with, but often labs will have legacy equipment running – either from different vendors or even from the same vendor but with different software platforms. As a data guy, a chromatogram from seven different vendors is seven different techniques. The outputs are different, the columns are different, the headers are different – everything is different. And that makes things much more challenging.
Nichola: That problem definitely resonates with me as well. In pharma, as we look to more and more challenging targets, the molecular complexity is increasing, we need to choose the instrument that is best suited for addressing our analytical needs, which often necessitates heterogeneity.
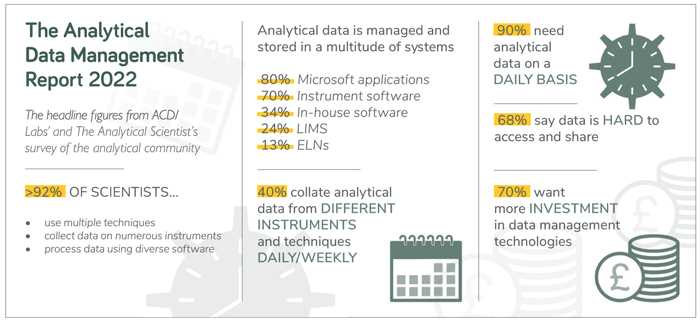
What’s the secret to a successful data management strategy?
Mark: Support from upper and middle management is absolutely essential. The people in the lab doing the leg work already have a great deal on their plates and data management does involve additional upfront work to create and fill in the data tags. So management will need to give people the extra bandwidth. But there also needs to be a bottom up understanding; what’s in it for the lab technicians? If you’re going to make someone fill in 10 fields on the front or back end, try to include some sort of automation so they’re no longer manually typing results or creating so many reports. The net result might be a similar overall workload as you move towards effective data management, which ultimately should make everyone’s lives easier.
Graham: I’d also suggest making a priority list. Ask yourself, what do we most need that we can’t access very easily right now? Then make sure that you’re thinking strategically about how to get from point A to B. That may seem trite, but it is important to focus on value for your organization.
Nichola: We need to see a transition from thinking of data as single-use pieces of information that are consumed and then no longer usable. Data itself can be an incredibly valuable resource, especially given the pace at which digitization, AI, and machine learning are advancing. So I think it’s really important to start your data management strategy with that mindset.
Are there benefits beyond efficiency?
Mark: Yes. I started down the digital journey as a lab manager, managing a team and dealing with chromatography and mass spectrometry, with purely selfish goals – making my job easier and improving team efficiency. But I discovered that there was so much more we could be doing with data. Now we treat data as an enterprise asset, like a reactor or a scientist, seeing how much we can squeeze out of it – reusing it and learning from it. For example, a report used to be something only a very small number of people with the right credentials could access in LIMS – the customer, perhaps a colleague of theirs, and the person on our end writing the report. Now, thanks to data management, scientists across the organization can learn from the data it contains.
Graham: This is a really good point that speaks to “data flow,” which is a real paradigm shift. Data consumers and data generators are increasingly seen as part of the same team – and they need to generate data that the organization considers an asset. Data management strategies have to adjust to this shift if companies want to handle their data most productively.
Having traveled your own data management journey, what would you do differently?
Nichola: It is easy to underestimate the amount of time you’ll need to properly dedicate to data management. For me, it isn’t my main job – I’ve got many other pulls on my time. So if you’re embarking on a big project like this, make sure it gets that management buy-in Mark mentioned!
Mark: Agreed. Plus, don’t underestimate the importance of harmonization. Labs in different countries might have different languages or date formats. But it’s not your job as the overarching person to dictate these things: the scientists in the different labs need to get together and figure out what works best for them.
Graham: I’ve learned to incorporate something we refer to as a “define and design” phase, which involves sitting down with a variety of stakeholders and addressing some of the kinds of issues Mark raised – discussing priorities and figuring out timelines. It can be at a high level, but you have to use real examples to make it concrete for people.
Dive deeper with Graham, Nichola, and Mark as they discuss challenges of analytical data management in this on-demand webinar.
Within AstraZeneca, we had two medicinal chemistry groups generating thousands of samples for testing with the task of selecting the best candidate drugs to take forward. We wanted a centralized solution, so we decided to go cloud-based and make that accessible across the organization. The solution includes data from different lab instruments, existing file shares on our lab servers, as well as the data our external collaborators share with us. That data is automatically uploaded to our cloud-based storage system, and, at that point, the relevant metadata is extracted from the data set.
We’ve got quite robust tagging – what the data is, where it comes from, who’s running it, what the sample is, and we use that to organize and search. The centralized cloud storage enables us to have multiple consumers of that data, whether that be lab chemists accessing the data or data scientists for certain ongoing machine learning projects.
On top of that, if the data meets the minimum metadata requirements, it’s also ingested into an ACD/Labs Spectrus database, which is where most of our analytical scientists access the data. We can search, visualize, and perform processing.
References
- ACD/Labs, The Analytical Data Management Report 2022. Available at: https://bit.ly/3ZNKQkf