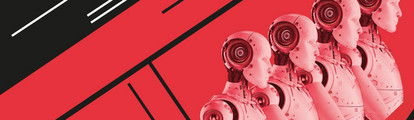
Do Androids Dream of Analytical Chemistry?
We ask Peter Harrington, Director of the Center for Intelligent Chemical Instrumentation at Ohio University, whether artificial intelligence can help solve the field’s big data challenges.
What is the current focus of your work?
We are working on spectroscopy and mass spectrometry analyses of botanical medicines, specifically cannabis. Our goal is to take the complete spectrum of a complex mixture, and relate that fingerprint to the chemical and pharmacological properties of the sample – known as chemotyping. To develop these complex algorithms, we use machine learning (also known as artificial intelligence).
One of the biggest challenges with natural medicine is biological variability. Everything from the growing conditions to the harvest date of the plant to how the plant material has been processed can affect the chemical composition; even samples from the same plant may have different properties. By chemotyping a sample, we get a snapshot of the overall chemical composition, which we can tie (directly or indirectly) to the properties that we’re interested in, such as physiological effects in the body. The alternative approach is to try to identify every compound (or the active compounds) in the mixture and quantify them, but that’s very time consuming and costly.
Longer term, we would like to see cannabis companies using the chemotypic data we’re generating on different strains to help direct people to the product best suited to them. At the moment, staff in cannabis dispensaries make personal recommendations to their customers – this strain for migraines; that strain for insomnia – but there’s no scientific basis; it’s all anecdotal. We’d like to get science back into that decision-making process. Keeping a history of each consumer’s experiences and correlating it with the chemical compositions of their chosen products would allow us to direct them to the products that they personally might find most effective. This kind of profiling might be applicable in the wider pharmaceutical industry too, where there’s still often a “one-size-fits-all” approach to usage and prescription. As people’s lives become increasingly digitized, we can use predictive algorithms to look for correlations between specific drugs and their effects on consumers.
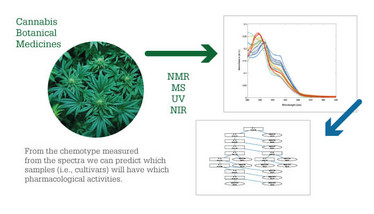
Our approach to identifying the chemistry behind specific pharmacological traits in cannabis.
How are you using AI?
Simple artificial neural networks have been used since the earliest computers were developed in the 1940s. Later, multiple layers of neural networks were combined to boost learning power – known as “deep learning”. We can see deep learning in action every day in applications like Apple’s Siri or Facebook’s face recognition feature.
Once the layers of neural networks get very deep, it becomes very hard to determine how the decisions are being made – in our case, to determine the relationship between the chemical signal and the property that we want to measure. To correlate peaks with properties, we need to be able to peel back the layers. We use an Enhanced Zippy Restricted Boltzmann Machine (EZRBM) developed by machine learning pioneer Geoffrey Hinton to modify the data structure so that we can determine what peaks correlate with which properties.
AI has a number of applications to problems in analytical science – particularly because it’s now so easy to collect large amounts of data from which computer algorithms can learn.
Is the technology available to handle so much data?
We go through a cycle in which computer technology and analytical instrumentation keep leapfrogging each other – one pulls ahead, and then the other needs to catch up. In analytical instrumentation, there are three things that we would like to improve. One is the use of automation – for instance, using autosamplers that can collect data 24 hours a day, seven days a week. The second is chromatographic resolution (meaning more data) and the third is time resolution (meaning the instruments run faster). All three will lead to more data per sample.
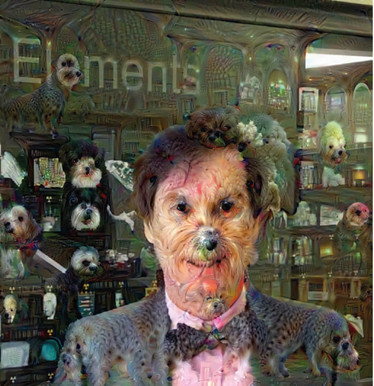
Google’s Deep Dream Generator (https://deepdreamgenerator.com) – trained on
images of the author’s dog – attempts to render his picture, with surreal results!
Then we have advances in computer technology. At the moment, these center on increasing our storage capacity. Twenty years ago, I gave lectures in which I tried to explain the number of connections in the human brain – about one terabyte – by saying that you would have to connect 1,000 one-gigabyte hard drives to obtain the technological equivalent. Nowadays, we can buy 10 TB hard drives for a few hundred dollars!
The next issue to tackle is locating data on a drive that size. That’s also improving; our scan rates are steadily increasing. So, whenever a scientist tells me that their computer is not large enough or fast enough to handle these amounts of data, I say, “That may be true now, but in two or three years, it is likely to be another story.”
What are the limitations of AI?
I find the rapid development of machine learning exciting – but people don’t always agree. They ask me, “Should we be afraid of advances in AI?” I don’t think so, although it’s not unreasonable to consider the disadvantages. Because these systems are so complicated, when things go wrong, they tend to go terribly wrong. And once they’ve gone wrong, they can be hard to fix. And there are other limitations; machine learning is just an algorithm that follows a procedure – it is not “intelligence,” just as a recipe is not a cook. A human being still has to write that algorithm and tell it what to do; we have yet to reach the point where computers can program themselves. They also can’t deal well with new information. You may have seen “computer dreaming,” which is a form of digital art in which you train a system using a series of images (for example, of dogs) and then provide them with new input (such as a picture of a human). The algorithm attempts to build a picture of a human using what it has learned about dogs, which, as you can imagine, is quite surreal. So, the technology is not without its problems, but I don’t think we have anything to fear!
A former library manager and storyteller, I have wanted to write for magazines since I was six years old, when I used to make my own out of foolscap paper and sellotape and distribute them to my family. Since getting my MSc in Publishing, I’ve worked as a freelance writer and content creator for both digital and print, writing on subjects such as fashion, food, tourism, photography – and the history of Roman toilets.
Peter de Boves Harrington is based at the Ohio University Center for Intelligent Chemical Instrumentation, Department of Chemistry & Biochemistry, Athens, Ohio, USA.