Enter the METRIX
We plug into the (RAMAN)METRIX with Juergen Popp, who discusses clinical spectroscopy’s exciting AI-enabled future
Markella Loi | | 5 min read | Interview
Spectroscopy has great potential to improve clinical diagnostics, but has yet to be incorporated into routine clinical workflows. Here, Juergen Popp, Scientific Director of the Leibniz Institute of Photonic Technology, Germany and chair for Physical Chemistry at the Friedrich-Schiller University Jena, Germany argues that the combination of multimodal spectroscopy and AI is set to change the face of clinical research.
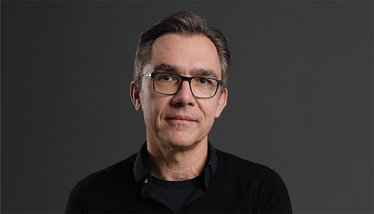
How could multimodal spectroscopy change clinical research?
Intelligently combining multiple spectroscopic methods leads to a significant improvement and increase in the density of information that can be obtained from spectroscopic data. Many existing needs in medical diagnostics cannot be met by a single modality and instead require the interplay of multiple spectroscopic modalities (one modality can often elucidate only a single detail).
Each spectroscopic modality has its strengths and its limitations, but what if we combined several techniques to exploit their best features? “Multimodal” spectroscopy enables the simultaneous production of signals for more than one spectroscopic modality, yielding improved acquisition speed, penetration depth, sensitivity and molecular selectivity.
Research in this field in recent years has demonstrated this impressively, and numerous proof-of-concept studies exist that show the added value of multimodal spectroscopy for improved medical diagnostics and therapy. A prime example – pursued in our group – is the use of nonlinear multimodal CARS/TPEF/SHG imaging to display the morpho chemistry of tissue for improved intraoperative diagnostics.
You have designed RAMANMETRIX for the automated analysis of Raman spectral data in clinical settings, how does it improve spectroscopic analysis?
In recent years, Raman spectroscopy has developed into one of the most powerful spectroscopic methods with an incredibly broad range of applications. Raman spectra – displaying characteristic frequencies of molecular vibrations – is a kind of “molecular fingerprint” of the sample under investigation that enables the identification of chemical molecules or changes in tissues or cell components caused by metabolic or pathophysiological processes.
Using machine learning-based approaches for an automated analysis of Raman spectra can enhance the process. However, Raman spectra of biological samples must be pretreated to remove interfering signals prior to the analysis of their Raman spectra. Working on this problem in recent years led to the development of a new software, RAMANMETRIX – an online platform for the automated analysis of biomedical Raman spectroscopic data.
What potential hurdles do you expect before AI-driven spectroscopy is more widely adopted?
The main obstacle in the wider adoption of AI-driven biomedical Raman spectroscopy is the optimal pretreatment of Raman spectra for model training. It is essential to adopt pretreatment routines generating Raman spectra for building a “generally applicable” model. In this context we made a big step forward by developing the online software platform RAMANMETRIX.
One big challenge comes down to the actual application of deep learning methods in Raman data analysis – specifically about the amount of Raman data available. Deep learning requires large independent Raman data sets, since small sample sizes lead to poor deep-learning algorithm performance. We also need to ensure the generalized ability of trained deep-learning-based models, meaning a model performing well on one dataset should not lead to a bad performance for another dataset. This again depends on the adoption of generalized pretreatment routines.
To overcome all these challenges, we must expand beyond proof-of-concepts studies for a rather limited patient cohort. For example, this can be achieved by initiating large open-source Raman datasets in an international ring trial study. The more popular Raman in medical diagnosis becomes – which is also triggered by advances in AI – the more Raman data are generated worldwide.
But the path of translating a research idea into a product is a rocky one, and here we must cross the so-called valley of death. We have now reached the stage where we can test our Raman approaches in clinical trials on a large cohort of patients. There are regulatory hurdles to overcome here, such as compliance with the EU Medical Device Regulation (MDR) 2017/745 in Europe, which is often difficult if not impossible for research institutions. Industry, on the other hand, often only gets on board once reliable data from such MDR-compliant clinical trials on many patients are available, clearly demonstrating the added value. This essentially creates a “vicious circle.”
Funding is needed here to enable solutions that have been verified as functional in principle to be further developed as medical devices. Only through such MDR-compliant research and development is it possible to translate research solutions into everyday clinical use and thus also later in industrial utilization.
Another prerequisite for promoting the translation of research ideas into medical products is special infrastructures that offer user-open platforms (for example, under the umbrella of a university hospital) and bundle the expertise of renowned players from science and industry to accelerate the translation of new diagnostic and therapeutic procedures in the long term. The Jena located “Leibniz Center for Photonics in Infection Research (LPI),” which was recently included by the German government in the national roadmap, shows what such translational research could look like in concrete terms. LPI will become a translation infrastructure that accompanies new solutions from idea to validation, while significantly shortening development time. LPI will become a translation infrastructure that accompanies new solutions from idea to validation, while significantly shortening development time – serving as a blueprint for other medical conditions, such as cancer and neurodegenerative diseases.
What other spectroscopic trends are you excited about?
The second quantum revolution by non-classical light sources is definitely an emerging approach I see becoming a trend. The measurability of new characteristics of a light field, beyond the intensity distribution, opens new conceptual and technological possibilities. The use of non-classical light (quantum entanglement) for linear and non-linear spectroscopy will allow researchers to exploit new temporal and spectral windows that cannot be reached with classical light. This will boost the sensitivity, selectivity and speed of molecular spectroscopy to new dimensions, allowing us to address questions that cannot be answered at the moment.
Additionally, the application of new materials, such as metamaterials or photonic nanomaterials, in spectroscopy would offer new possibilities in terms of boosting, for example, non-linear light matter interaction down to a single molecule level with highest spectral and temporal resolution.
Image Credit: Supplied by Interviewee
Associate Editor, The Analytical Scientist