No Prize for Second Place?
Rather than rushing to the finishing post, we should all focus on data quality by sticking to the four Cs: consistency, correctness, completeness, and credibility
Meriem Gaida |
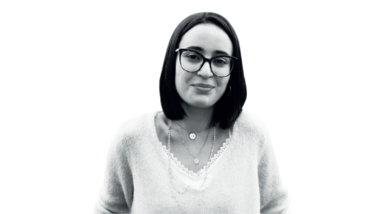
What does it take to provide good quality data in science? A rudimentary question and yet a profoundly important one – especially for young emerging scientists at the start of their careers.
In a world bouncing between two paces – quick and quicker – our self-worth can often be driven by our ability to produce and to deliver. And though this is a natural human reaction, one should still keep in mind that faster does not always mean better – particularly in science! We must ensure that we’re producing high-quality data and not producing data for its own sake.
Good quality data entails good lab practices, and reflects the researchers’ attitude, commitment, and ethic. As researchers, we are expected to critique our own research and the research of others. We must ask relevant questions before starting any project to make sure we avoid common data pitfalls.
How can I certify the quality of my data? Well, it is simple! The data needs to satisfy the “four C’s”:
- Consistency: compatibility within each collected data point and suitability of the dataset they form for the research question.
- Correctness: the dataset contains no aberration and is relevant to the value that is measured.
- Completeness: the produced dataset does not contain missing values.
- Credibility: the produced dataset is plausible and realistic.
The temptation to overlook one or more of these rules of thumb can be strong – especially when up against a deadline and intense competition for grants and academic positions. Unfortunately, tweaking study results to achieve a statistically significant outcome is not an uncommon practice in science. In fact, according to a Nature survey, 70 percent of researchers did not manage to reproduce other scientists’ experiments and 52 percent of the participants to the survey attest to a significant reproducibility crisis in science (1).
Some practices are far too common: cherry picking, ruling out data that do not seem to reinforce the starting hypothesis; P-hacking, testing, arranging, filtering, tweaking and/or tuning of the dataset to obtain a statistically significant result; and outcome switching, altering a protocol to rule out inconclusive or negative results. The data must lead to the conclusion and not the opposite!
Recently, a Nature investigation (2) raised concerns about the manipulation of the publishing process via “paper mills” – firms that produce falsified research. The study revealed that in January 2021, 68 papers were retracted from the RSC advances journal due to allegations that they may be linked to paper mills. By March 2021, 1300 articles were identified as being fraudulent and 26 percent of them were already pulled back or stamped with expressions of concerns. This comes with no great surprise since the number of retracted articles had increased 10-fold during the previous 10 years, with fraud accounting for about 60 percent of these retractions (3). In a Nature statement, Elsevier and other publishers expressed their deep concern over paper mills, saying that what they are currently witnessing is only the tip of the iceberg – but that they’re doing their best to combat falsified research. Many journals are now implementing a stricter review process by demanding that the editors ask for the raw data and by training and hiring people to stamp out suspicious manuscripts.
Managing data responsibly and with integrity makes findings easier to share, reuse, and, most importantly, to reproduce – and data reproducibility is a cornerstone of good quality research. Finally, good quality data helps scientists improve their visibility and facilitates collaborations. It can also help speed up innovation and, perhaps even more importantly these days, restore the public’s faith in science.
Just remember: science is not meant for quick fixes!
- M Baker and D Penny, “Is there a reproducibility crisis?”, Nature, 533 (2016). DOI: doi.org/10.1038/533452A.
- H Else and R V Noorden, “The fight against fake-paper factories that churn out sham science”, Nature, 591, 516-519 (2021). DOI: doi.org/10.1038/d41586-021-00733-5
- J Brainard and J You, “What a massive database of retracted papers reveals about science publishing’s ’death peanalty’”, Science, (2018). DOI : doi: 10.1126/science.aav8384
PhD student in the Organic and Biological Analytical Chemistry (OBiAChem) laboratory, University of Liège, Belgium