Agrochemical Analysis at the Edge
Given the rising global demand for food, are the analytical tools, techniques and methods at our disposal up to scratch?
Silvio Vaz Jr. | | 4 min read | Opinion
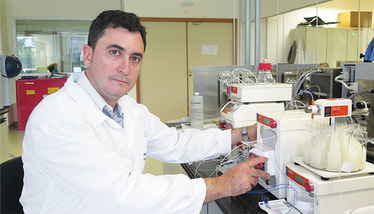
Agrochemicals are an important family of biologically active molecules used in industrial agriculture. This sector generates a diversity of analytical matrices with their own physical characteristics, which demands a range of advanced analytical techniques and methods for monitoring and quality control of processes, raw material, inputs, and products.
Due to their potential to negatively impact the environment and public health, agrochemicals must be carefully monitored and controlled. With that in mind, what is the cutting edge of agrochemical analysis – and are they up to scratch?
There are several agrochemical classes comprising herbicides, fungicides, insecticides, nematicides, and plant growth regulators (1). The kinds of molecules (or active ingredients) used in each of these classes depends on climate conditions (tropical or temperate), the crop type (corn, soybean, fruits, and so on), and the pest incidence (insects, diseases, and so on).
The end-product (or formulation) is composed of the active ingredient (generally considered the analyte) and variable quantities of additives (for example, polyglycerol ester, anionic emulsifier, non-ionic emulsifier, mineral oils, dimethylamide derived fatty acids) – the sum constitutes the analytical matrix. These additives can turn into analytes if we need to perform, for instance, chemical analysis to determine impurities (2).
Agrochemical formulations are generally produced in liquid, solid or a mixture of both physical states in the same ending-product (for example, liquid dispersions), as wettable powders, capsules, and emulsions. In some situations, independently of the physical state, derivatization may be necessary when using chromatography after the extraction step.
In other words, agrochemical matrices represent high complexity, which also often demand suitable sample preparation methods, of course.
Several advanced analytical techniques are already being used for agrochemical analysis. For example, for quantitative information, chromatographic techniques (both gas and liquid phases) are hyphenated with several detectors, including atomic emission spectrometry, optical emission spectrometry, atomic absorption spectrometry, and ultraviolet-visible absorption spectrophotometry. For qualitative information, absorption infrared spectroscopy, nuclear magnetic resonance in solid and liquid states (13C and 1H nuclei), mass spectrometry, Raman spectroscopy, thermal techniques (DSC and TGA), particle size distribution, and zeta potential are all used.
These techniques can be applied for a large variety of analytes and analytical matrices for chemical analysis of agrochemicals, depending on the application. Regulatory guidelines set limits of detection or quantification, or maximum residue limits – but issues related to costs, infrastructure, and availability of skilled labor also determine which method is used.
And that’s possibly why artificial intelligence (AI) and chemometrics have emerged as important auxiliary tools in agrochemical analysis. AI can be used as a machine learning tool to extract insights from large and intractable data sets, as well as aiding in the automation of repetitive tasks (3). AI can also help make process analytical technologies (PAT) more scalable and applicable. There is room for improvement here – and I anticipate growth in the number of available advanced software and systems for laboratory automation and control.
Chemometrics does not only apply to measurements, but also to the extraction step of analytical processes because it allows users to evaluate the effect of operational parameter variation on the recovery percentage values. It is possible, for example, to compare several extraction methods and choose the most suitable one for a given group of analytes or to assess the effect of the matrix on the analyte group across methods. Furthermore, chemometrics can be combined with machine learning, as an AI tool, to monitor synthetic processes. Chemometrics for data treatment can be allied to machine learning for several spectroscopic techniques (for example, Raman spectroscopy, NMR, and XRF) to reach a better understanding of generated data (4).
Overall, agrochemical analysis is a fascinating branch of analytical chemistry, involving a large family of analytical techniques for several matrices and methods. And it is also a growing branch of analytical chemistry – in terms of scale, scope and importance – given the rising global demand for food, which is set to grow by 35–56 percent by 2050 (5). Analytical techniques and methods can help food safety by guaranteeing the absence of harmful bacteria, viruses, parasites, or chemical substances – and promoting sustainability in agriculture and livestock.
I believe this class of biologically active molecules can be well-monitored and controlled by the analytical instrumentation available today – provided those tasked with monitoring and controlling agrochemicals have complete access. Unfortunately, this isn’t a given in many parts of the world. Furthermore, I strongly recommend agrochemical analysts apply the principles of green chemistry in their analytical processes to reduce waste, use safer solvents and auxiliaries, and reduce derivatives. There is room for improvement here – and, given the size of the agrochemical market, widespread adoption of eco-friendly analytical chemistry could have a major impact in the 21st century.
Image Credit: Supplied by Author
- S Vaz Jr, “Introduction to Sustainable Agrochemistry”, Sustainable Agrochemistry, Springer: 2019. DOI: 10.1007/978-3-030-17891-8_1.
- OECD, “OECD Test Guidelines for Chemicals” (2023). Available at: https://bit.ly/3LZLIxm.
- K Baum et al, “From responsibility to reason‑giving explainable artificial intelligence,” Philosophy & Technology, 35, 12 (2022). DOI: 10.1007/s13347-022-00510-w.
- R Houhou and T Bocklitz, “Trends in artificial intelligence, machine learning, and chemometrics applied to chemical data,” Analytical Science Advances, 2, 128-141 (2021). DOI: 10.1002/ansa.202000162.
- M van Dijk et al., “A meta-analysis of projected global food demand and population at risk of hunger for the period 2010–2050,” Nature Food, 2, 494–501 (2021). DOI: 10.1038/s43016-021-00322-9.
Research Scientist at Brazilian Agricultural Research Corporation, Parque Estação Biológica, Brazil.