Deep Beer Wins
How machine learning outperformed traditional analysis of beer flavorings – laying the foundation to tailor foods with superior qualities
Markella Loi | | 7 min read | News
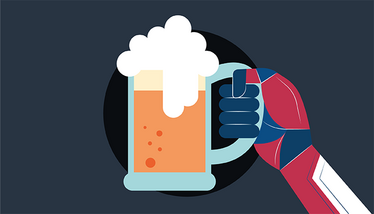
Food and beverage industries rely on human taste for quality control and product development – and, in most cases, this means that every single batch is examined by a trained tasting panel and can be subject to personal preferences. Why? Nothing beats a trained tongue (and nose) – until now. An interdisciplinary research team from Belgium have combined analytical methods with sensory science and artificial intelligence (AI) to chemically profile 250 different beers – creating accurate flavor prediction models (1).
To learn more about the study and its potential impact, we sent questions to first author Michiel Schreurs (Junior PHD Researcher, VIB–KU Leuven Center for Microbiology, Belgium) and corresponding author Kevin Verstrepen (Professor in Genetics and Genomics at Leuven University, Belgium).
What are some of the current challenges in this field?
The reliance on humans is a big challenge, because the training and maintenance of those panels is very expensive, but they also struggle with consistency and repeatability (human tastes evolve over time and are influenced by things like our mental state). The best way to ensure consistent results is by using bigger panels, which come with bigger costs.
Our senses of aroma and taste are highly developed, we are extremely good at tasting foods. Although it feels very natural to taste foods, the flavor is actually very complex. Simple foods contain dozens of flavor-active chemicals, and for complex foods like chocolate, coffee and beer, this runs up into the thousands. How our senses detect all these flavor chemicals and how our brains interpret them into flavors is very complex, and, up until now, there is no machine that can do the same task.
How does your recent research on beer flavoring address such obstacles?
The chemical measurement of flavor-chemicals has improved massively in recent decades. At the same time, there has been a revolution in artificial intelligence (AI), and we now realize that computers can learn extremely complex processes. Even though we often cannot understand exactly how the computer achieved its goal, we can use these computers to make predictions.
We believe that combining our modern analytical tools and AI can finally help us bridge the gap of flavor, so we can reduce the reliance on human tasters – or at the very least assist the tasting panels. On top of that, we think AI can be a helpful tool for product development, guiding food manufacturers in the steps they can take to help improve the flavor of their foods.
How did you conduct this study?
We used multiple techniques to characterize different classes of compounds. We employed gas chromatography (GC) to detect volatile aroma compounds and identify common flavor compounds like alcohols and esters. We then performed an untargeted analysis using GC-MS – which enabled us to profile the chemical compounds we were measuring as outcomes of malts, hops, and fermentation. Finally, with enzymatic and spectrophotometric approaches, we measured the most abundant flavor compounds, like ethanol, sugars, proteins, glycerol, and the bitter-tasting compounds in beer. This combination of analytical techniques gave us a broad overview of the volatile and non-volatile flavor compounds that play a role in beer.
To collect the sensory data, we took into consideration our tasting panel’s ratings – focusing on approximately 50 flavor descriptors (bitterness, sweetness, fruitiness, maltiness) – and consumer data from the online beer review website RateBeer. The ratings on this platform gave us data about what the average consumer thinks of a beer – which doesn’t always correspond to trained panels. The latter is often more critical of flavors that are missing or that should not be present, while online reviewers are influenced by price, brand name, and other reviewers.
What is the role of machine learning in your research?
Machine learning is the tool that allows us to connect our chemical dataset to our sensory dataset. The power of machine learning is that it can look at the effect of everything together; in other words, it can take all our chemical measurements (over 200 different compounds) and see how they influence flavor. Instead of being limited to a few compounds, we get a much fuller picture – and that’s really crucial when it comes to flavor; we know that flavor compounds can interact with each other, with some flavors amplifying each other and others hiding (“masking”) specific flavors.
What were the main findings from your research?
The biggest result is that machine learning outperformed the conventional tools that are used in food science today. It showed that our idea has merit: given the right data, computers can learn and predict how foods (or at least beer) will taste.
We also showed that you can ask the computer what its predictions are based on. The computer can list the chemical compounds that it deems important for a specific flavor, which can lead to new insights into how flavor works. We asked the computer model which compounds it used to predict a high appreciation of beers. When we added these compounds to a commercial beer, our panel actually found it to be better in a blind tasting.
You can imagine that such a task is not so straightforward for humans. For instance, can you tell me which chemical compounds make you like strawberries? Trained humans can sometimes do this for a select number of well characterized flavor compounds, but for most flavors this is incredibly difficult or impossible. The computer, on the other hand, can easily list the chemical compounds that it deems important.
What does your study mean for manufacturers and consumers?
For manufacturers, it opens up a lot of new possibilities. First of all, they can look into cost-saving by reducing their dependency on tasting panels. But machine learning might even help to streamline their process in general, detecting that things are going wrong before humans can. Furthermore, this approach can lead to new insights into how flavor works. This knowledge can guide manufacturers with their product development.
For consumers, the benefits will be in the product itself. They can expect even better tasting foods, including healthier foods that taste as good as the less healthy alternatives (think alcohol-free drinks tasting exactly like their alcoholic counterparts or plant-based foods tasting much more like meat). Meanwhile, manufacturers can try to tailor products to specific groups of consumers, meaning the foods you buy are more suited to your liking.
Consumers might feel skeptical about AI-driven food tailoring. How do you plan on addressing such criticism?
It’s important to keep in mind that we are in no way taking humans out of the equation. Instead, this technology is just another step towards more streamlined production and development. I like to compare it to the invention of temperature regulation, which massively improved all types of food production, but definitely did not replace the bakers and brewers. These new models will help manufacturers obtain the products that they already have in mind, giving them a faster way to achieve the foods that they want to develop. There will always be people making the changes (taking into account the computers suggestions) that allow us to make improved foods.
We actually have received a lot of positive feedback after our publication. I expected more criticism, but it seems like consumers are more eager to embrace these technologies than we first thought.
Finally, what is next for you and your research?
In our paper, we focused on improving appreciation because it is the most complex (and personal) flavor attribute – people can agree that something tastes bitter, but that doesn’t imply they both like it. By showing the potential for this very complex flavor trait, we show the potential for all.
Now that this has been done, we don’t think “improved appreciation” is the best way forward. Flavor appreciation is very personal, cultural, regional, and is best solved with an approach that incorporates this (like identifying specific groups of consumers and tailoring products to them). We will leave that to the food manufacturers!
Instead, we like to focus on specific flavors. We recently built models to predict the “alcoholic” taste of beers, with the goal of improving non-alcoholic beers. Of course, ethanol was very important there, but our models also revealed a number of alternative flavor compounds that shape the alcoholic taste. We are now testing different combinations of these by adding them to non-alcoholic beer, and so far, the results look very promising! We hope that this research can help make non-alcoholic beers better – more similar to regular beer – so that consumers are more likely to choose this healthier alternative.
- M Scheurs et al., Nat Commun, 15, 2368 (2024). DOI: 10.1038/s41467-024-46346-0.
Associate Editor, The Analytical Scientist