The ‘One Pot’ Approach
Tom Metz, Integrative Omics Scientist and Metabolomics Technical Lead at Pacific Northwest National Laboratory (Washington State, USA), selects six papers that exemplify the power of multi-omics.
Tom Metz |
When people select one technology over another it’s usually because they “grew up with it”, which is either dependent on where they did their PhD work, or which kind of -omics they happened to apply first to their area of biology. So many molecules are detected with -omics technologies that the false positive rate is likely higher than we expect given today’s tools and metrics. When basing subsequent hypotheses and publishing results on single-omic studies, there is bound to be misinformation put forth. Being able to perform additional -omics experiments will help constrain that to some extent. For example, if someone performs a transcriptomic study and has complementary proteomic data (or other -omics data), they will be able to check if what they thought might be going on at the transcript level had propagated through to the protein or the metabolite level.
Pieces of the puzzle
For one thing, transcriptomics doesn’t give you any information at all about post-translational modification of proteins, such as phosphorylation and signaling; the only way to capture such information is to do the appropriate proteomics analyses. If resources are not a limitation, then I would suggest that a multi-omics approach should be taken (when reasonable). Clearly, it depends on what questions are being asked, but if the questions are open-ended, then the more data you have, the better.
Here and there, multi-omics is taking the place of a single -omics. You can tell how seriously we take it at PNNL – we have an integrative -omics group! The Department of Energy Office of Biological and Environmental Research has funded many large programs to study both isolated microorganisms and microbial communities. The goal of these programs is to achieve a systems-level understanding of these organisms and communities such that they could be engineered or otherwise manipulated for the benefit of society, such as for improved biofuels production or carbon sequestration. And the National Institute of Allergy and Infectious Diseases (NIAID) has sponsored the Systems Biology for Infectious Diseases Research Program since 2008. We have been part of that program since its inception and (like others who were funded) have been using transcriptomics, proteomics, metabolomics, and lipidomics to study pathogenic bacteria and viruses. I would say there have been enough papers using multi-omics approaches for me to describe it as in vogue...
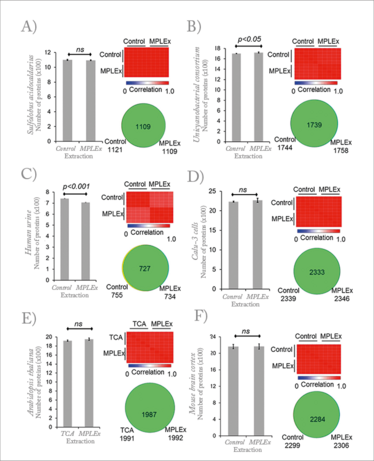
Figure 1. Proteomic coverage, number of identified proteins, and correlation over five replicates for MPLEx and control for different samples. A) The archaeon S. acidocaldarius. B) Unicyanobacterial consortium. C) Human urine. D) Human lung epithelial cell line Calu-3. E) A. thaliana plant leaves. F) Mouse brain cortex. Abbreviations: MPLEx: metabolite, protein, and lipid extraction; Control: no-extraction control; TCA: trichloroacetic acid extraction. Taken from paper 1.
Omic efficiency
In essence, with a “one-pot” sample extraction, you’re going to save time and effort – one of the big drivers for me and my collaborators in the NIAID systems biology project. It’s also likely that it reduces overall experimental variability, because you’re no longer trying to integrate data that came from separate cells – you’ve now got protein, metabolite and lipid data from the same cells. If you were to include a step to extract the genetic material, as others have done, then you could also combine the DNA/RNA data sets.
With microbial communities, for example those bacteria that reside in soils and particularly in association with plant root nodules, the scientific community in general is realizing that we need to get beyond 16S sequencing to discover which organisms are there, and instead focus on what those organisms are doing metabolically. That’s not only what metabolites they might be producing and releasing into their respective environments, but also what proteins they’re producing and how the microbiota are interacting with each other and their environments, including any hosts. This is also the case for the gut microbiome. Could metabolites and proteins that might be released in the lumen of the gut act as signaling or hormone molecules and affect the health of the host? There have been many very cool studies showing that certain populations of microbiota are associated with certain diseases. Now, we need to mechanistically understand why particular phenotypes are associated with those populations of microbiota – and that means looking at the genes that are being expressed, and the proteins and metabolites that are being produced. It’s a very complex problem – but multi-omics is best suited to unraveling all of these questions.
Papers pushing multi-omics
- ES Nakayasu et al., “MPLEx: a robust and universal protocol for single-sample integrative proteomic, metabolomic, and lipidomic analyses”, mSystems, 1, 1-14 (2016).
- H Roume et al., “A biomolecular isolation framework for eco-systems biology”, The ISME Journal, 7, 100-121 (2013).
- SC Sapcariu et al., “Simultaneous extraction of proteins and metabolites from cells in culture”, MethodsX, 1, 74-80 (2014).
- SA Schmidt et al., “Two strings to the systems biology bow: co-extracting the metabolome and proteome of yeast”, Metabolomics, 9, 173-188 (2013).
- J Tisoncik-Go et al., “Integrated omics analysis of pathogenic host responses during pandemic h1n1 influenza virus infection: the crucial role of lipid metabolism”, Cell Host & Microbe, 19, 254-266 (2016).
- L Valledor et al., “A universal protocol for the combined isolation of metabolites, DNA, long RNAs, small RNAs, and proteins from plants and microorganisms”, The Plant Journal, 79, 173-180 (2014).
- W Weckworth et al., “Process for the integrated extraction, identification and quantification of metabolites, proteins and RNA to reveal their co-regulation in biochemical networks”, Proteomics, 4, 78-83 (2004).
Being an experimentalist at heart, I appreciate those multi-omics papers that focus on methods for “one-pot” preparations of samples to enable extraction of all the molecules necessary for multi-omics analyses. Because my primary area of research is metabolomics and lipidomics, I’ve chosen the papers that go beyond genes and proteins to cover metabolomics, lipidomics and other small molecule data. Transcriptomics and proteomics are more mature, whereas metabolomics and lipidomics are still developing and evolving to become more robust. I like it when investigators try to bring in additional data on other small molecules. Why? Even though it’s riskier (because the platforms are less mature), I don’t think it is appreciated just how valuable such information can be.
Waste not, want not
For a long time, we’ve used a protocol to prepare metabolites and lipids from samples by using a mixture of organic solvents. A liquid-liquid bilayer of polar metabolites and non-polar metabolites (which are the lipids) forms, and then precipitated proteins are located in the middle after centrifugation. In metabolomics studies, we would discard the protein because we weren’t necessarily doing proteomics. I figured that was wasteful and wondered if we could use the precipitated protein for proteomic analyses. I wanted to make sure that we could get just as good data with the precipitated protein as we would with a traditional proteomics preparation. And so we went through a pretty extensive literature search to see who had done it before, in what context, and with what biological samples. We found that there had been initial demonstrations of using precipitated proteins, but no one had really assessed the reproducibility or quality of the proteomics data. It had also never been applied very broadly – so we thought we’d give it a shot. Of course, we could have been even broader in the sample types and conditions we investigated, but again we’re always limited by perhaps the most important -omics of all: economics! The funding only goes so far... In any case, the result of our efforts was MPLEx (metabolite, protein, and lipid extraction, see Figure 1).
We found that precipitated proteins, for the most part, give proteomic data that is comparable with the data obtained from working up proteins using traditional methods. And it turns out that the coefficients of variation (CV), which is a measure of reproducibility, are just as good as the standard approach – sometimes better. We found statistical differences in certain cases, but that was almost assuredly due to the nature of the proteins in those particular sample types. We’re certainly very comfortable using the approach to gather multi-omics data. Certainly, our MPLEx method is not the only way to gather all the molecular types needed for multi-omics – and I welcome other investigators to rise to the challenge by adopting or adapting other protocols.
The next step is to also add the genomics data. The authors in the ISME paper – H Roume et al. – included a pre-step where they could isolate genetic material (DNA and RNA). And the paper by W Weckworth does the same for one of the plant samples. I see no reason why one cannot get all the molecular types necessary for a complete multi-omics study from DNA all the way down to metabolites. I believe that the main limitations are the resources and technical skills that the investigator has at their disposal.
Tom Metz is Integrative Omics Scientist at Pacific Northwest National Laboratory, Washington, USA. His research has focused on the development of both global and targeted metabolomics and lipidomics capabilities based on LC and gas chromatography (GC) coupled with mass spectrometry, both for fundamental studies of metabolism/metabolic interactions as well as for biomarker discovery.