An Automated Avenue for Microplastic Detection
An AI–spectroscopic synergy could be the key to identifying microplastics faster and with more accuracy
Jessica Allerton | | News
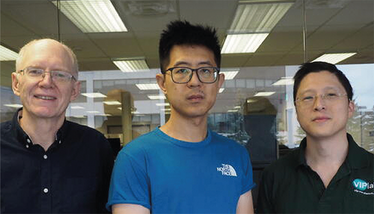
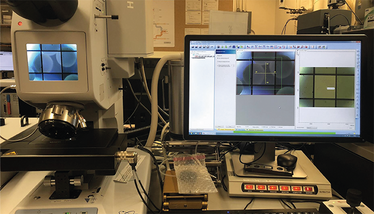
An AI-based microspectroscopy imaging identification system, PlasticNet, can detect microplastics with high accuracy – and could be used in wastewater treatment and food production plants (1). The tool, developed by researchers at University of Waterloo, Canada, uses a deep learning convolutional neural network architecture to automatically detect images generated by focal plane array (FPA)-based micro-fourier transform infrared (FT-IR) microscopy.
After training PlasticNet with over 8,000 spectra of virgin plastic, and then retraining the tool using non-virgin plastics, PlasticNet was able to successfully classify 11 types of common plastics with 95 percent accuracy. The team also demonstrated a 17.3 percent improvement in identifying polypropylene microplastics compared with other environmental datasets.
“I was surprised by the efficiency of deep learning in this context,” says lead author Ziang Zhu. “With PlasticNet, we received precise and reliable results in a field that is known for often dealing with complex and variable data. It’s really opened up avenues for future investigations in environmental science.”
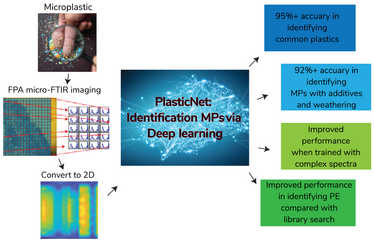
After trailing PlasticNet at a local wastewater treatment plant, the team are confident in taking their discovery to the next step. “Our findings mark a significant step forward in environmental research,” says Zhu. “PlasticNet’s ability to rapidly and accurately recognize microplastics could pave the way for a standardized method in micro and nano pollutant detection.”
Zhu is hopeful that environmental researchers will embrace AI for detecting microplastics – as well as other pollutants and applications: “Without acceptance of this technology, we’re unable to apply it to the complex criteria involved in different areas of environmental science.”
We hope you found this article informative! Why not join our mailing list to receive new insight every week? Subscribe here
Image Credit: Ziang Zhu
- Z Zhu et al., ScienceDirect, 337 (2023). DOI: 10.1016/j.envpol.2023.122548.
Associate Editor, The Analytical Scientist