Omics Shines in 2024
Looking back on 12 months of mass spectrometry-based omics breakthroughs
James Strachan | | 6 min read
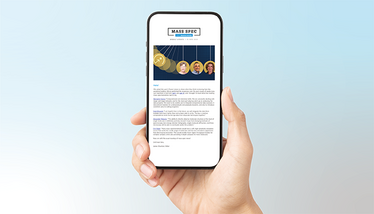
As we reflect on the past 12 months of research covered in the Mass Spec Newsletter, it’s clear that 2024 was a standout year for the mass spectrometry-based omics community. To celebrate these achievements, we’ve rounded up the breakthroughs that truly captured our attention.
If you attended ASMS this year, you may have noticed the plethora of talks and posters on single cell omics – the same was true in the Newsletter. For example, in March, Melanie Bailey and colleagues developed an untargeted single-cell lipidomics method based on microflow chromatography coupled to a data-dependent mass spectrometry method for fragmentation-based identification of lipids. Later in the year, Mayo clinic researchers used single-cell and single-nuclei proteomics to understand cellular heterogeneity, including post-translational modifications and variants that cannot be achieved through single-cell genomics.
But it was Jonathan Sweedler’s single-cell metabolomics neuroscience breakthrough from February that really caught our eye. Sweedler and his colleagues from the Beckman Institute for Advanced Science and Technology introduced MEISTER – an integrative experimental and computational mass spectrometry framework for imaging and chemical profiling of single cells. MEISTER comprises deep-learning-based signals, a multimodal image registration technique that produces coherent 3D reconstruction of MSI data, and a computational approach to create and map cell-specific chemical profiles for multiscale integration.
The researchers successfully created a 3D map of the rat brain with high spatial resolution (50-μm lateral and 16-μm sections) – profiling 13,566 single cells and extracting lipid profiles from 11 brain regions. “We envision future endeavors on creating multiscale biochemical atlases, with increasingly powerful profiling technology for metabolites, lipids, peptides and proteins, as well as integrative analysis with other omics data,” concluded the authors in their paper.
Continuing with metabolomics, the Mass Spectrometry Search Tool (MASST) returned – and it’s better than ever…
The web-enabled mass spectrometry search engine was firstly introduced by the Dorrestein lab in 2020 – enabling its users to search a specific molecular “fingerprint” against spectral data that have been deposited in one of the largest public repositories for mass spectrometry data in the world, GNPS/MassIVE.
However, limitations – particularly in untargeted metabolomics for microbial analysis – remain; “A single search with the original MASST would take around 20 minutes, making it impossible to programmatically search for hundreds or thousands of MS/MS spectra, and the obtained output was not easily interpretable because it was not associated with metadata. So, we developed microbeMASST – an evolved and domain specific version of MASST,” says Simone Zuffa, microbeMASST creator and postdoc scholar at Skaggs (you can read the full interview from July here).
Moving on to a story that shook up the metabolomics field; in June, Martin Giera, Aries Aisporna, Winnie Uritboonthai, and Gary Siuzdak published a study suggesting that the metabolome isn’t so dark after all. The team mined the METLIN MS/MS database – consisting of more than 930,000 molecular standards – at 0eV, an energy designed to simulate the absence of collision-induced dissociation. They found that in-source fragmentation could account for over 70 percent of the peaks observed in typical LC-MS/MS metabolomic datasets.
The Analytical Scientist Presents:
Enjoying yourself? There's plenty more where that came from! Our weekly Mass Spec Newsletter brings you the most popular stories as they unfold, chosen by our fantastic Editorial team!
We spoke with Giera and Siuzdak to find out more about the implications of their recent discoveries:
“Previously, the assumption was that all detected peaks represented intact molecules (as most networking approaches do). This leads to erroneous network interpretations due to the inclusion of data from these in-source fragments artifacts,” they say. “Our study highlights the necessity for more selective data interpretation, ensuring that only real molecules are considered. This shift will enhance the accuracy of metabolic networks, avoiding distortions from non-existent molecules and providing a clearer, more reliable map of metabolic interactions.”
In more metabolomics mayhem, scientists at Van Andel Research Institute, USA, identified more than 1,000 previously undetected proteins in common metabolite samples; these persist despite extraction methods designed to remove them. The authors believe the findings could have “broad-reaching implications across all research fields using metabolomics and molecular metabolism.” In the paper, the team provides a practical solution to mitigate the problem using 3 kDa filtration. "Ensuring that our phenotypes are free of any confounding proteins is critical to attaining accurate results,” said corresponding author Ryan Sheldon in a press release. “Our findings help us do just that."
Now, from the metabolome to the proteome – a one hour human proteome, to be precise. The Coon Lab in collaboration with Orbitrap pioneer Alexander Makrov and his team published a paper describing the “deep analysis of the human proteome in less than one hour.”
The research team employed a variety of analytical tools – including the latest version of the Orbitrap Astral mass spectrometer – to perform proteome characterization. In a 30-minute period, this MS-based approach enabled the identification of 10,411 human protein groups on average. The authors conclude that their study “is ushering in a new era of proteomics where a harmony of throughput, depth, and sensitivity are enabling unprecedented studies of biological impact.”
That brings us to Nature’s Method of the Year 2024: spatial proteomics. A global team led by Matthias Mann made a significant discovery in the treatment of toxic epidermal necrolysis (TEN) – an often fatal and previously considered untreatable condition that causes widespread detachment of the skin.
The new approach, dubbed deep visual proteomics (DVP), combines ultra-sensitive mass spectrometry with microscopy and AI-driven analysis to study specific cell types from tissue sections to an unprecedented degree of detail. Using DVP, the team identified the hyperactivation of the inflammatory JAK/STAT pathway in samples from patients suffering from TEN, establishing JAK inhibitors as a potential treatment option. When put in practice, JAK inhibitors produced consistent and overwhelmingly positive results.
“Spatial proteomics represents a powerful new way to understand disease mechanisms at molecular resolution,” said first author Thierry Nordmann in a recent interview with The Analytical Scientist. Our work shows how this technology can make a real difference. We were able to analyze thousands of proteins in specific cell types from archived patient samples, discover a key pathway driving disease, and translate this finding into successful treatment.”
Finally, I’d like to highlight several more research stories from the first half of 2024 related to disease diagnosis and treatment. In January, researchers from the Baylor College of Medicine set out to find an alternative – combining mass spectrometry-based proteomics with their newly developed kinase inhibitor pulldown assay (KiP). The optimized methodology enabled accurate and simultaneous measurement of a 100-strong panel of target kinases. “This paper emphasizes that new methods in protein mass spectrometry hold great promise for better definition of the individual druggable landscape present in each cancer and should be more widely used for research and, ultimately, clinical care,” said corresponding author Matthew Ellis in a press release.
In February, researchers combined metabolomics with machine learning to diagnose ovarian cancer with 93 percent accuracy. In March, scientists conducted the first in-depth MS-based proteomic analysis of isolated extracellular vesicles – identifying ovarian carcinoma-specific proteins. In April, a platform combining MS-based proteomics and machine learning enabled the identification of prognostic biomarkers in colorectal cancer patients. In May, multiomics blood-based biomarkers were used to predict Alzheimer’s predementia with high specificity in a multicentric cohort study. And finally, Heck Lab researchers used proteomics and complexome profiling to evaluate in a year-long study longitudinal variations in the plasma proteome of kidney failure patients, prior to and after a kidney transplantation.
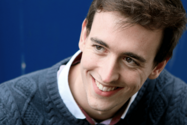
Over the course of my Biomedical Sciences degree it dawned on me that my goal of becoming a scientist didn’t quite mesh with my lack of affinity for lab work. Thinking on my decision to pursue biology rather than English at age 15 – despite an aptitude for the latter – I realized that science writing was a way to combine what I loved with what I was good at.
From there I set out to gather as much freelancing experience as I could, spending 2 years developing scientific content for International Innovation, before completing an MSc in Science Communication. After gaining invaluable experience in supporting the communications efforts of CERN and IN-PART, I joined Texere – where I am focused on producing consistently engaging, cutting-edge and innovative content for our specialist audiences around the world.