What got you interested in AI?
I’ve spent the past 35 years as a chromatographer and mass spectrometrist, searching for new enabling technologies and producing learning and development materials for analytical scientists. My first introduction to “AI” in the form of large language models (LLMs) was via my son – a physics undergraduate – who claimed, “ChatGPT has revolutionized my Python coding and problem solving.” This was around 18 months ago and was enough of a prompt for me to dive a little deeper into ChatGPT 3.0 – the latest version of the model at that time. Candidly, after a little “exploration,” I chose not to pursue the LLM research further, as the initial results were disappointing – probably due to my inexperience and lack of time.
Roll forward six months and I was tasked with writing some “stand out” job advertisements for two roles within my department and, for some unknown reason, I once again turned to an LLM to see what help it could be. The result was amazing. It produced very concise but unusually phrased adverts from a list of keywords that I provided. I decided to go with the results, totally unedited. One month later we had appointed two very capable candidates who are showing great promise in their early career!
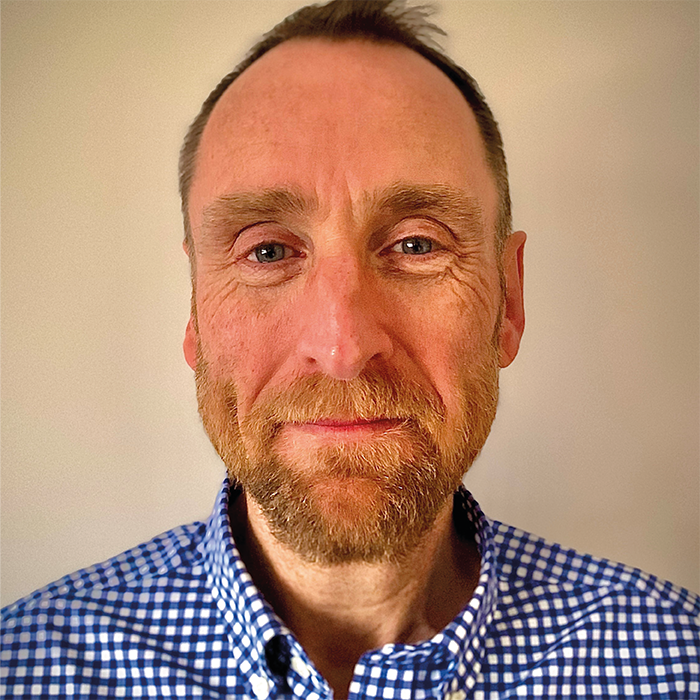
So I decided to give ChatGPT (1) another shot. I started by asking ChatGPT how it can help chromatographers. It said:
- Educational resource
- Troubleshooting assistance
- Interpretation of results
- Experimental design
- Keeping updated with current research
- Safety and best practices
- Integration with laboratory systems
- Collaborative brainstorming
- Enhancing public understanding
- Continuous learning
How can ChatGPT help chromatographers as an education resource?
Let’s put it to the test, shall we? To test the Educational Resource benefits of ChatGPT-4, I provided the following prompts:
- Can you explain the advantages of SPME Arrow versus standard SPME techniques?
- Compare the efficiency of 2.7um superficially porous particles and 1.7um fully porous particles in HPLC
- In what types of analysis can the use of ion mobility filtering improve LC-MS analysis?
- Suggest an appropriate approach to optimize a GC-MS analysis using a Design of Experiments approach
i. Can you suggest a fractional factorial design for the critical variables listed previously
ii. How would I use ANOVA to analyze the data from the fractional factorial design experiments
As you will already know from the nature of the above questions (selected from topics that I’ve been recently discussing), the answers could all have been gleaned from web searching. What is different about the LLM responses is that they are summarized and are an indicator of where one may want to undertake more specific web-based research or to ask the LLM more specific questions. In essence, the responses gave a good distillation of reasonably wide topics, acting as a jumping off point for more in-depth learning.
For Question 4, you’ll notice that I delved a little further with more specific questions relating to the initial response, which suggested some of the variables that one may want to include in the experimental design. The responses were pleasing, yielding a fractional design table over 64 experiments (there were a large number of variables) and the ANOVA response suggested an experimental approach and some statistical programs that could be used to undertake the regression analysis. This was a good demonstration of the uniqueness of the “transformer” architecture of these highly trained neural networks in which the order of words (information) and contextual analysis of the sentence (structure) can lead to seemingly more specific insights and can concatenate knowledge over multiple subject areas to give the impression of intelligence. The responses elicited here appeared to have an appreciation of how one might specifically undertake experimental design for my hypothetical GC-MS analysis.
Here though, I’d like to highlight a concern that I will develop further as we progress. In truth, with over 35 years experience in chromatography, I have a fair appreciation of what the “correct” answer is; I have a large amount of contextual intuition to help sense when something isn’t quite right. I worry that less experienced chromatographers may be overly reliant on the responses being correct, without the wider frame of reference or the benefit of experience.
What about as a troubleshooter?
I asked ChatGPT-4 the following questions to investigate the model’s chromatography troubleshooting capabilities.
1. What might cause a quadratic response for trace analysis in GC-MS? (Answer: 504 words)
i. Can you refine your answer knowing that the analyte in question is Bis (2-ethyl hexyl) phthalate (DEHP)? (Answer: 475 words)
ii. Could you rank the previous suggestions from most likely to least likely cause (Answer: 275 words)
iii. Could you recommend a strategy to reduce DEHP contamination (Answer: 473 words)
2. Both chromatograms show the same gradient analysis. Both are blank samples. The bottom chromatogram is after 10 injections of blank solvent. Why are there so many more peaks than in the first injection?
i. I use HPLC grade acetonitrile and 18 megohm resistivity water for my mobile phase - what impurities may be present that would give rise to peaks such as these?
3. Can you tell me the problem with these HPLC Chromatographic peaks?
i. Could you refine your response given that the sample diluent is 100% acetonitrile?
ii. What if my analyte is only sparingly soluble in water?
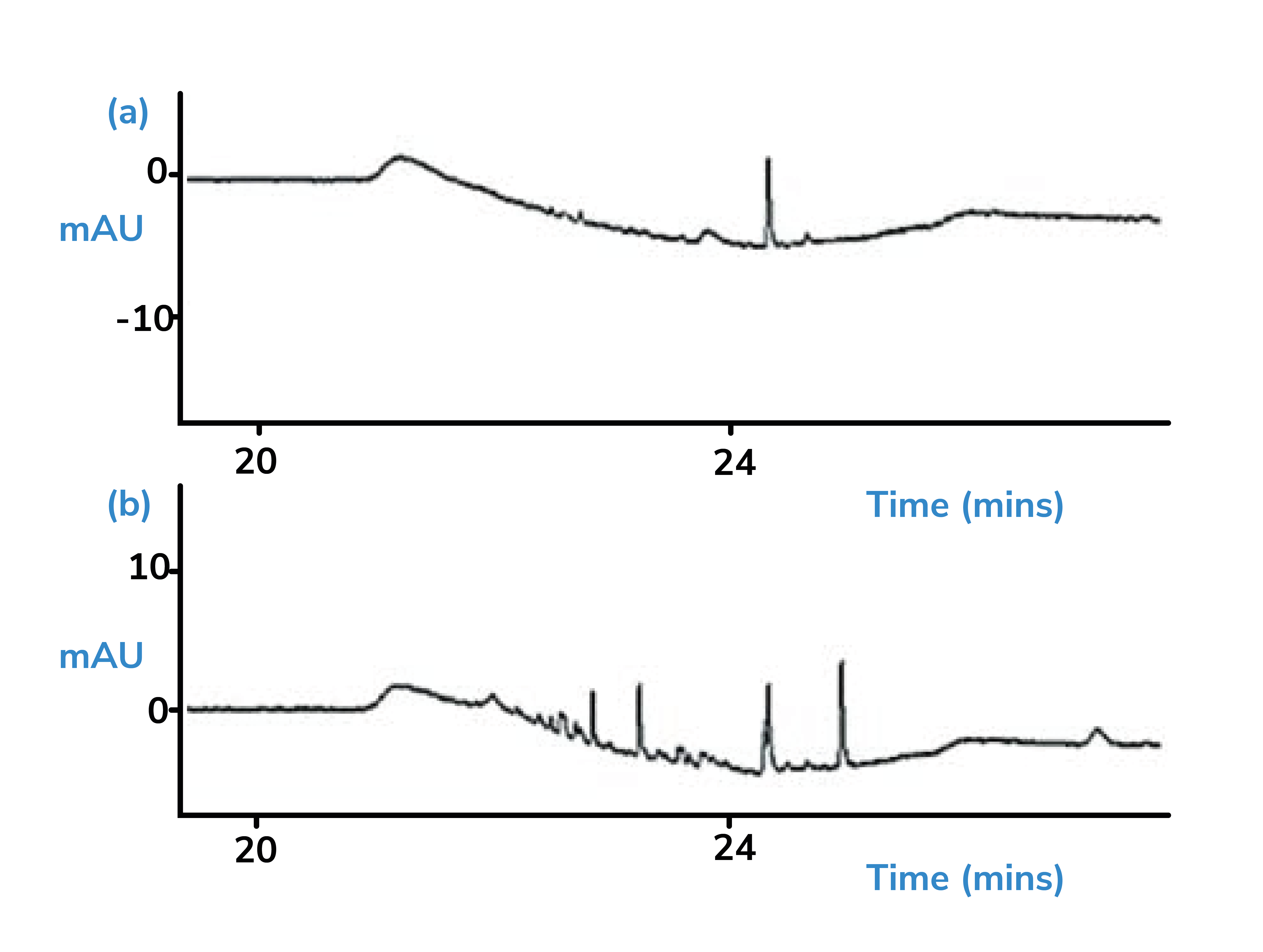
Image reproduced with permission, www.CHROMacademy.com
4. What might be the cause of very low sensitivity for ethyl acetate in electron ionization GC-MS?
i. How can I optimize the GC-MS parameters, especially the ion source and detector settings be optimized to improve sensitivity for ethyl acetate?
ii. From literature, what electron energy is typically used for ethyl acetate GC-MS analysis?
iii. What would happen to the ethyl acetate sensitivity if I reduced the ionization source to produce 20 eV electrons?
iv. I use an Agilent 5977B GC-MS - what ion source parameters can I optimize to improve sensitivity for the ethyl acetate analysis?
v. Can you give me a literature reference for the analysis of ethyl acetate by GC-MS?
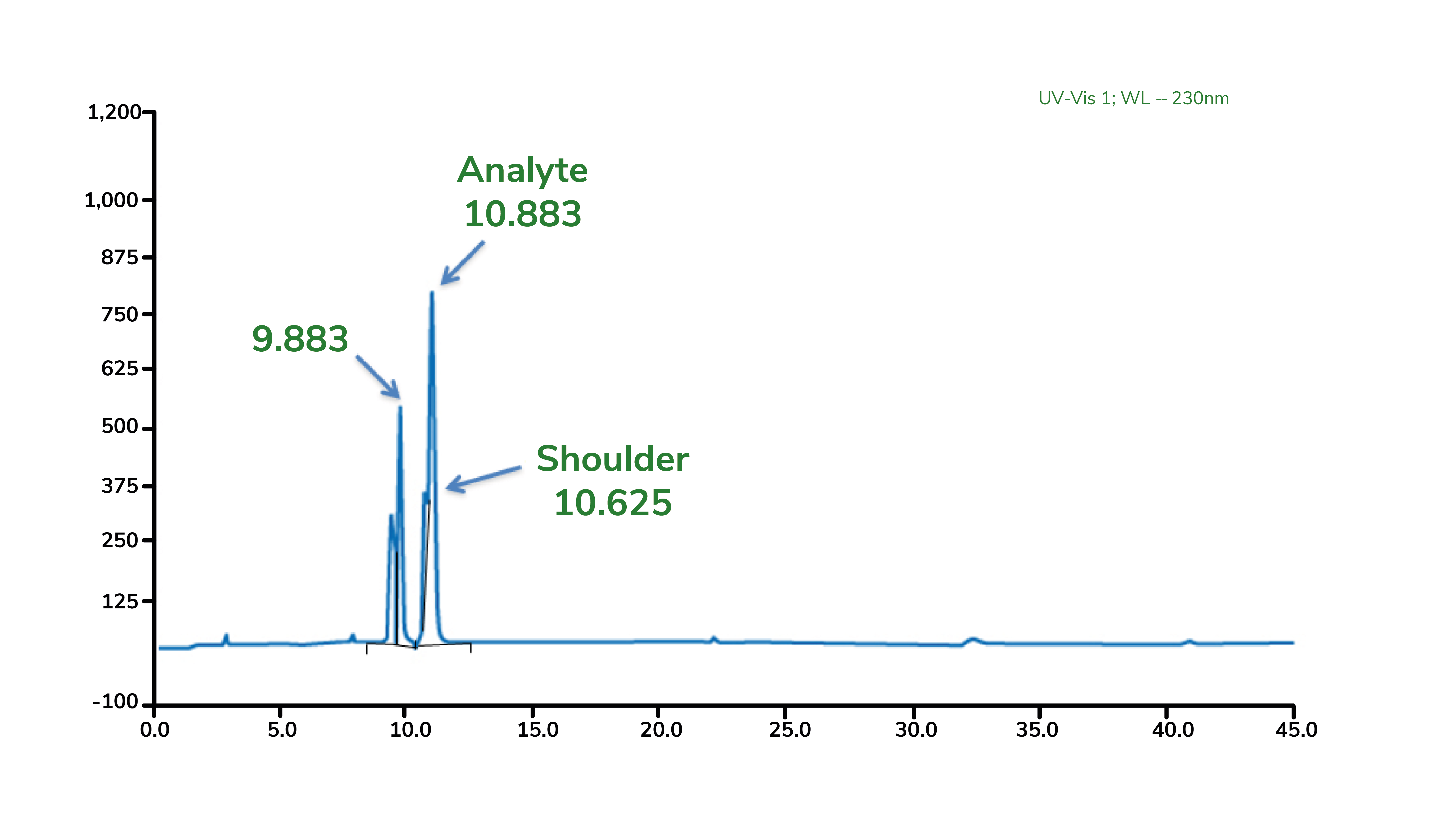
Image reproduced with permission, www.CHROMacademy.com
I hoped to present the LLM with a range of different troubleshooting scenarios and was immediately struck by the “conversational” style of the interactions. It felt like honing a browser search where one might refine search terms based on the results of the previous search; however, these interactions were considerably quicker and more focused – typically I was asking for a refinement, clarification or prioritization based on the previous answer.
Overall, I have to say that I’m really very impressed with the quality of the responses. I’m absolutely blown away with the ability to interpret graphical images – this was simply stunning and a total revelation in comparison to previous versions of the ChatGPT that I’ve used.
As you can see from the word counts against the responses in Question 1, the suggestions do tend to be quite wordy; and, more generally, responses all tend to be somewhat generic. Sweeping statements, with caveats urging me to consider the particular analysis in question or contacting your instrument vendor, seem to be standard form.
However, for Question 1, I did arrive at a fairly good set of suggestions for reducing DEHP contamination in the laboratory; and although other plausible causes of non-linear response were suggested in the initial response, I decided to push the questioning down the contamination route. It also did a pretty good job of ranking the potential sources of contamination and suggesting ways to reduce contaminant levels. Again, Question 2 was answered very well and although the first response was very broad, my clarifying question produced a solid list of tasks to consider to reduce the contamination/carry-over which was correctly identified from the chromatograms. Ranking and possible ways to reduce contamination were again very plausible. In Question 3, the responses were very good indeed, the system correctly identifying split peaks and associating these with a variety of causes including diluent/eluent eluotropic strength mismatch. When pressing for answers based on real world sample solubility issues, it was able to give some reasonable suggestions on how to solve the problem. Whilst Question 4 was reasonably answered, the responses never really arrived at the answer I was looking for, although admittedly I did try a curve ball with the follow up questions around reduced electron energy!
Overall, I feel a little like I’m in a conversation with a knowledgeable but slightly “waffly” friend. The issue remains that, given my experience, I feel like I know this friend well and can tell when they are bluffing or being too vague and can probe their knowledge with a few more targeted questions. If I didn’t know them well, and hence didn’t trust them (analogous I think to a less experienced chromatographer), I’d find it difficult to sort the bluster from the golden nuggets of information.
I feel I’m selecting specific information from each response to further develop the arguments, which needs a certain amount of context. Without this, I think my new “friend” may be slightly too obtuse to be incisively useful. However, the information produced is useful as a starting point for further research or troubleshooting activities, especially given the engines ability to rank or prioritize actions based on contextual information.
As a very simple test of the system’s ability to interpret data I asked the following questions – I’ll pre-empt the discussion by saying that, though these were very simple questions, things didn’t go well…
0.1 1 5 10Std. Concentration Response 101242 1084845 5997498 10810348
1. Can you calculate the slope and intercept of this data?
i. Are you sure this correct – I get a different answer using Excel
ii. These answers are still very different to Excel – can you troubleshoot your calculation?
iii. Nope still different – can you try again please?
iv. Still different – I used the LINEST function in Excel to derive my data, how are you getting different answers from this?
v. Results from Excel were slope 1090722 an intercept 108327
2. Can you predict the isotopic distribution for the GC-MS molecular ion of 1-(1-Naphthyl)-2-propanamine?
3. Can you predict the GC-MS spectrum for 1-(1-Naphthyl)-2-propanamine?
4. What type of compound might give rise to a GC-MS spectrum with ions as m/z 77, 91, 93, 121?
5. What significance does an ion with m/z 91 have in GC-MS spectra?
Responses to Question 1 were quite alarming. The explanation of the rationale and methodology (including equations) was very helpful. However, as you will see from the question structure, I was given five different responses, all of which were incorrect and the final response could be summarized as “trust your Excel results because everyone uses it.” The conversation revealed another worrying aspect of this LLM in that it is very quick to “apologize and correct.” If I’m to build trust in a relationship, the ease with which a different answer is proposed makes me very uneasy about any information previously proposed.
Without laboring a point, there really wasn’t anything useful in the responses to questions 2 to 5, however I would urge the reader to try these out and see if you can discern anything usable. I’m “looking for answers” rather than judging how useful the information could be to a “newbie” in the subject.
How can ChatGPT help curate research?
My final areas of interest were Experimental Design, which I’m interpreting as Method Development, and Keeping Updated with Current Research. Conversations (I’m learning not to call them questions) were as follows:
1. Suggest conditions for the headspace extraction of residual acetoin and acetic acid from water by headspace GC-MS
i. Acetoin and acetic acid have similar polarity to water, won't the sensitivity of this method be very low?
ii. What about using the salting out technique to increase sensitivity in headspace – can you recommend a salt to use and at what concentration?
iii. Would direct injection of water into a GC cause problems?
iv. Can you recommend some water compatible GC columns?
In the interests of brevity, I only asked one question of this type. Honestly, I didn’t need to ask any more because I could see exactly which way this was going. It’s back to the “waffly friend” syndrome; however, this time I do feel they were genuinely trying to help – to the point of being overly apologetic sometimes when they felt they weren’t “pleasing” me with their responses (oh my goodness – I’ve already started to anthropomorphize the LLM…).
Objectively, I do believe that there was a lot of useful information that could be gleaned regarding the general approach to methods such as this. But I did need to “guide” my friend along the way and, honestly, I’d have been better simply looking up a manufacturer’s application note.
2. What are the pKa values of glycine?
3. Which manufacturer has the most applications for the chromatographic analysis of PFAS in air?
4. Can you recommend chromatographic conditions for the analysis of PFAS from ambient air?
5. What is the LogP value for glycidol and what source do you get the information from?
6. What HPLC column could I use to retain uracil?
Yes, I could have looked most up most of these things using a web browser, but I wanted to see if the LLMs were going to offer me anything above and beyond the simple web search. Broadly, I’d say not, but see my later comment on other LLMs that I’ve begun to investigate. I was perhaps most disappointed with the responses regarding the PFAS application. The model didn’t seem to be able to access manufacturers literature and cited that its “cut-off” date was January 2022, so presumably anything after this time would not be captured.
In terms of Keeping Updated with Current Research, given the forgoing discussion, I wasn’t holding out a lot of hope. The conversation with the LLM was:
1. What is the latest Literature Research for the analysis of Nitrosamines in Drug Product?
i. Can you cite any academic literature titles which discuss Nitrosamines analysis?
I can keep the discussion short here – these questions resulted in absolutely nothing useful. Merely a list of likely literature sources (PubChem and so on) and an apology that the LLM did not have access to real time data or provide specific academic literature titles. I wonder then how the claim of ChatGPT-4 being able to help us keep up to date with Current Research could be substantiated?
Let’s talk about AI life beyond ChatGPT-4... Are there other AI tools out there?
Yes, there are some other AI tools that I have begun to investigate lately. The first is ChemCrow (2). It is very new to me and a model that I haven’t had time to fully explore, but it does appear to hold a lot of promise. The product connects LLMs such as ChatGPT-4 with a wide number of chemistry expert tool application programming interfaces (APIs) to ease the programming burden for the analytical chemist. Expert tools that can be queried using a natural language style including molecule naming and exact molecular weight from SMILES strings and vice versa, product price and availability from SMILES strings, identification of CAS numbers from molecule names, Tanimoto similarity between molecules, the ability to modify molecules by generating forward and retrosynthetic rules, and patent checking and identification of functional groups from SMILES strings. Primarily used to date for the prediction (and automation) of synthetic routes, there is some useful functionality within the model for the analytical chemist. I accessed the model via a free interface called “huggingface” (3), which gives web interface access to a limited number of the tools and functionality, and I have used it to produce molecular structures, SMILES strings, and a wide range of physico-chemical descriptors – all very useful when planning and troubleshooting separations.
Of course, there are programs available that can do much of what I have used ChemCrow for, but the ability to use natural language queries and to interact and refine the conversation is very useful. Clearly, from the Python code developed and shown within many of the responses, those with some programming knowledge would find this platform very useful. The model is open source and also presents the user with code for a python “operating” environment – again very useful for the modern analytical chemist with some programming knowledge.
I have also recently been using OPSIN (4) – a web-based Parser for Systematic IUPAC nomenclature that seamlessly generates molecular structures from IUPAC compliant chemical names. I’ve also used SciSpace Copilot (6) – an excellent LLM tool to analyze scientific journal papers for content, impact, methods used, data produced, conclusions, and so on. Although it doesn’t seem to have access to all of the chromatography journals, it has enough access to be very useful when evaluating whether a particular paper is worthy of purchase or access via a library subscription. Further, it can import and parse previously purchased papers, and it can quickly summarize the content for rapid understanding and results interpretation. It is a very useful tool for keeping up to date with current research and perhaps filling the void that ChatGPT-4 seems to leave in this area. I was certainly able to gain some useful information regarding the latest research in the areas of PFAS and nitrosamines analysis from SciSpace Copilot.
Clearly there is a big divide between the highly accessible LLMs I have been using and the marvelous AI engines that are designed and implemented for the analysis of large datasets, experimental optimization, and interpretation and deconvolution of highly complex MS signals or nuclear magnetic resonance spectroscopy signal interpretation. These specialist tools are outside of my experience and understanding at this time, but are widely used and are developing rapidly. Instead, I have attempted to show tools that are easily accessible to the average analytical chemist (me!) and to explain the usefulness of these tools at the present time.
Any final thoughts on the future of LLMs for chromatographers?
In some areas, I have been really surprised by the usefulness of LLMs, especially in the areas of improving fundamental understanding and troubleshooting separations via the ability to upload problematic chromatograms and derive ranked pointers for possible solutions.
I believe it is reasonably widely understood that the popular LLMs, such as ChatGPT-4, have training datasets that are inadequate to be fundamentally impactful in terms of domain expertise or insight. The models do not currently contain or interface with “expert” computational tools; however, as I’ve described above, this may well be changing with initiatives such as ChemCrow.
Is AI in the form of the LLM my new best friend as a chromatographer? I would say not, but there are some features that I’m beginning to find quite endearing – who knows how our relationship may develop in the future!
Tony Taylor is Chief Scientific Officer, Life Sciences EMEAA, at Element Materials Technology, UK
References
- OpenAI, accessed between October 5 and October 30 2023. Available at: https://chat.openai.com.
- AM Bran et al., "ChemCrow: Augmenting large-language models with chemistry tools," arXiv preprint arXiv, 2304.05376 (2023).
- Huggingface, accessed between September 15th and October 28th 2023. Available at: https://huggingface.co/spaces/doncamilom/ChemCrow.
- OPSIN (Open Parser for Systematic IUPAC nomenclature), accessed between 5th and 30th October (2023). Available at: https://opsin.ch.cam.ac.uk/.
- SciSpace Copilot, accessed between 19th and 28th October 2023. Available at: https://typeset.io/.