Imaging Reveals Key Prognostic Markers in Head and Neck Cancer
Machine learning meets single-cell imaging to reveal cancer progression markers
| 2 min read | News
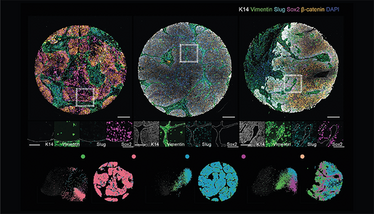
Three different so-called patient-specific fingerprints
Credit: Sara Wickström
A new imaging technique can identify distinct phenotypic "fingerprints" in head and neck squamous cell carcinoma (HNSCC) patients. The method combines machine learning with single-cell imaging to analyze the interaction between cancer cells and surrounding tissue at unprecedented resolution.
“This is a breakthrough in understanding cancer development and diagnostics,” said Sara Wickström, a research director at the University of Helsinki and senior author of the study, in a press release. “For the first time, we have shown that specific combinations of malignant cells and tissue cell types in what is considered healthy tissue have a strong prognostic effect on cancer progression.”
Using spatial transcriptomics, the research team, which included collaborators from the University of Turku and the Max Planck Institute for Molecular Biomedicine, mapped gene expression within the tumor and stromal compartments, while single-cell image analysis allowed for the precise visualization of morphological and functional differences within individual cells and tissues.
The analysis showed that the tumor-stroma interplay has a powerful impact on disease prognosis. Notably, patients with tumors characterized by a partial epithelial-mesenchymal transition (pEMT) phenotype – where epithelial cancer cells gain certain connective tissue features – had markedly different outcomes based on the type of surrounding stroma. Patients with pEMT tumors surrounded by cancer-associated fibroblast (CAF)-enriched stroma had significantly poorer survival rates, with five-year survival dropping to 21 percent, compared with those whose tumors were embedded in immune-enriched stroma, which showed a 90 percent survival rate.
Their analysis also revealed that specific signaling interactions, particularly involving amphiregulin (AREG) and the epidermal growth factor receptor (EGFR), play a critical role in driving cancer aggressiveness in pEMT tumors. AREG-EGFR signaling, often upregulated in CAF-enriched stroma, activates pathways that enhance the invasiveness of pEMT cancer cells. Since EGFR inhibitors are already used in some HNSCC treatments, targeting AREG-EGFR signaling in CAF-rich tumors could potentially improve therapeutic outcomes for these high-risk patients.
“In addition, our method was able to identify patients with a particularly poor prognosis who would benefit from an aggressive treatment strategy,” said Karolina Punovuori, a postdoctoral researcher at the University of Helsinki and co-author of the study. “On the other hand, we also identified a group of patients whose prognosis was good and for whom a less aggressive treatment, such as a surgical procedure alone, could be sufficient.”
Based on these phenotypic fingerprint findings, the researchers are developing a diagnostic test that could become a standard tool in clinical oncology, guiding treatment decisions for HNSCC patients. The team has also received funding to explore applications of this method in diagnosing other cancers, such as colorectal cancer.
“Our research utilizes the latest analysis methods in machine learning and spatial biology. We analyze hundreds of patient samples and millions of cells, which is only possible with the help of high-performance computers and artificial intelligence,” Wickström explained. "We believe that this technology will significantly improve cancer diagnostics and the accuracy of treatment strategies."