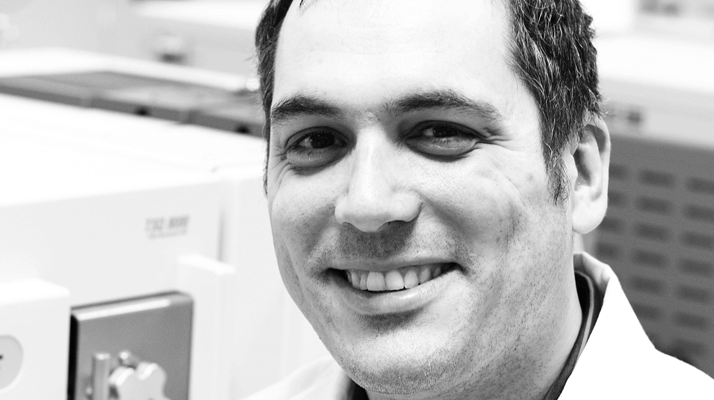
Believe it or not, I started out as an undergraduate in computer science and cybernetics. Unfortunately, the world of robotics involved a lot more mathematics than I expected. And so after a year of computer programming, I switched to pathobiology. But I never lost my interest in computers and programming, and that has been invaluable as I’ve progressed through my career; during my postgrad days I moved into bioinformatics and molecular modeling, which brought my two halves together. I soon realized that I wanted more time in the lab, which led me to do a research-based masters degree in biological and biomedical science. I ended up in the proteomics lab at the University of Glasgow in a world where robotics, wet-lab work, biology and computer happily co-existed. I’d found my calling – at least for a while. Using mass spectrometry coupled to computational techniques that make sense out of the biological data is where my broad interests now lie. In many cases, it’s not about creating new algorithms, it’s about processing data and presenting them in a useable format that biologists can understand.
So, why the shift from proteomics to metabolomics? One of the reasons was to get my hands on an Orbitrap instrument to be honest... I actually started out uncomfortable with the idea of metabolomics – it’s a completely different ball game. In proteomics, we could use Mascot to provide a probabilistic score for a given protein based on the mass spectra. You can use a cut-off system and, much like a court of law, you end up with an innocent or guilty verdict on the identity. In metabolomics, we were working entirely on mass and retention time – it’s a very binary way of working and felt quite limiting; it was a “yes” or “no” answer to identification without knowing how certain you were in either case. Now, we’re building fragmentation libraries and the requirements for supporting metadata in studies are increasing all the time. Indeed, metabolomics is now very rigorous – and it’s been a big learning curve for me in terms of quality control. Excellent reproducibility is key; dozens of replicates may be necessary to get the statistical quality for quantitation. And that’s the point where clinicians start to become very interested – robust, quantitative data on biomarker-style molecular relationships they are used to working with. I did most of my PhD work on a relatively fast-scanning but pretty low-resolution ion trap instrument. When I first got an Orbitrap instrument (an XL), I was showing my boss the data at 100,000 resolution, and he actually thought it was centroided – I had to zoom in about 20 times before I could demonstrate the reality of the peak widths. It was a really great moment! I’ve also done some work on high-resolution QTOFs, but stability of mass accuracy was a problem. The Orbitrap has always been rock solid in that regard. In fact, when we bought our ex-demo XL, it had been boxed up in the demo lab, left in a crate for three months, unboxed outside the building and bumped up a rough slope into the lab. After pumping the instrument down we found that it was still within 3ppm...