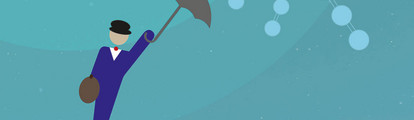
(Practically) Perfect Predictions
Chromatography method development is a time-consuming trial-and-error process. Is there an easier way?
When a separation scientist is asked to develop a new separation method for a group of target compounds, there are many decisions to make. What type of separation will I use (reversed-phase LC, hydrophilic interaction liquid chromatography, ion-exchange)? What stationary and mobile phase? What about the precise separation conditions?
The usual first step in answering these questions is to consult the literature in the hope of finding conditions under which at least some of your target compounds can be separated. If the literature is silent, the scientist must examine the structures of the target compounds and then rely on prior training and intuition to deduce the approach most likely to be successful. Normally what follows is a trial-and-error process, where different chromatographic conditions are explored until eventually the desired separation method is developed. As many readers will know from experience, this can take a great deal of time and effort.
For the past five years, my team has been working on a project that seeks to put method development onto a more objective and structured scientific footing. By examining retention databases of numerous compounds under different chromatographic methodologies and conditions, we can derive a mathematical “quantitative structure–retention relationship” (QSRR) that links some “molecular descriptors” (characteristics) of the database molecules with their retention behavior. The descriptors may be physico-chemical properties calculated from the structure (such as hydrophobicity, polarity, partition coefficient, and so on) or theoretical properties calculated using molecular modeling – a task that can be facilitated by molecular modeling software; for example, Dragon or Volsurf+. Such software packages use high-level computational chemistry theory to compute a large number of parameters from all aspects of a molecule’s structure.
The endgame? To develop a QSRR that can accurately predict the retention behavior of completely new compounds. The project runs across a team of five researchers (two postdoctoral fellows and three PhD students) at the Australian Centre for Research on Separation Science (ACROSS) at the University of Tasmania, supported by industrial collaborators. Funding was provided jointly by the Australian Research Council (ARC) and a number of industrial partners.
It’s complicated
We use the term “Holy Grail” to describe the goal of accelerated and reliable method development based only on chemical structures because it could potentially create a seismic shift in the way separation methods are developed. After all, chromatography is an exceedingly complex process that is governed by many experimental variables and, as a such, has long been considered an experimental discipline. Indeed, most separation scientists assume that its complexity prevents us from predicting retention behavior based on chemical structure. However, if one accepts a 5 percent error, the prediction of retention moves from theory into the realms of feasibility. Of course, a 5 percent error is too high if you wish to come up with the final optimal details of a new method – the best column, the precise mobile phase composition, the best flow-rate, and so on. However, chromatographic method development can be considered a two-step process, consisting of “scoping” and “optimization.” Scoping involves defining the broad characteristics of the separation, such as the type of chromatographic method, the type of stationary phase, the nature of the mobile phase, and perhaps even the approximate mobile phase composition. By contrast, the optimization phase involves finding the precise details of the method. Here, we are using QSRR only for scoping, where a 5 percent prediction error is sufficient to predict the broad characteristics of the best chromatographic method for the separation of a specific group of target molecules with known chemical structures. Final optimization of the chromatographic method will always involve detailed experimentation.
Of course, QSRR is not new – it has been applied previously by several research groups around the world – rather, the novelty in our approach (and perhaps the key to our success) lies in improving the manner in which the best compounds to derive the QSRR mathematical model are selected from the compounds listed in the database.
Relationship building
It has been a steep learning curve but by bringing together talented people with complementary skills covering chromatography and molecular modeling, we have made excellent progress. Indeed, we have all been pleasantly surprised with how accurately we can predict retention times from chemical structure. The key breakthroughs were the decisions to focus on local modeling rather than global, and to use concepts of structural and chromatographic similarity to select the best database compounds to train the QSRR model.
Allow me to briefly explain some of the terminology and how we came to our decisions.
First, QSRR models can be global or local: a global model is when one QSRR mathematical model is derived for all compounds in the database (put another way, all retention time predictions are made from the same global equation). By contrast, a local modeling approach gives each compound its own mathematical model equation. Predictably (no pun intended), the latter approach is more time consuming, but also much more accurate.
Choosing the best database compounds to train the QSRR model is both important and complex. Intuitively, one would expect that, if you wished to develop a local QSRR model for a target compound, then the best results should be obtained using only “training” compounds that are “similar” to the target compound, rather than using all the database compounds to train the QSRR. There are two types of similarity that are relevant to this clustering approach. The first is structural similarity and the second is chromatographic similarity.
As the name suggests, structural similarity can be calculated by comparing structural elements (functional groups, carbon chains, and so on) between molecules. The Tanimoto Similarity Index (Ƭ) is a frequently used parameter to calculate structural similarity and provides a number between 0 and 1 to describe similarity. It is relatively straightforward to calculate the pairwise Ƭ value between a target compound and all the database compounds and to then cluster only those compounds in the database for which Ƭ exceeds a chosen value (0.7, for example).
Chromatographic similarity clustering refers to finding those compounds in the database that show similar chromatographic behavior to the target compound. Typically, this means those compounds in the database that have similar retention times to the target compound. This type of clustering is paradoxical because to find the chromatographically similar compounds in the database, one needs to know the retention time of the target compound. Of course, this retention time is unknown and is exactly what the QSRR process is trying to predict!
Fortunately, we have discovered clustering tools that first use the Tanimoto Similarity Index but then also identify a surrogate compound (instead of the target compound itself) to use for subsequent chromatographic similarity clustering. Such a “dual” clustering approach has been shown to reduce prediction errors by up to a factor of 10 compared with no database clustering.
The QSRR approach we have developed is summarized in Figure 1.
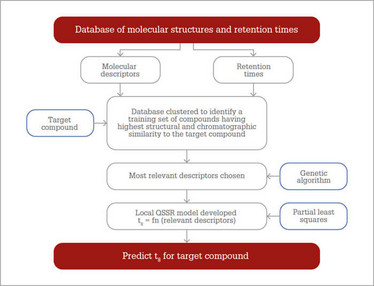
Figure 1. QSRR approach.
We start with a database of compounds with known structures and known retention times under specific chromatographic conditions. The aim is to have i) the largest database possible and ii) for that database to contain compounds with a wide structural and chromatographic diversity, so that, when clustering takes place, there will be sufficient compounds in the cluster to form a good training set. Molecular descriptors are then calculated for each database compound to give a array of descriptors with a matching retention time. The target compound is selected and the database is subjected to dual clustering to find a training set comprising the compounds with the highest structural and chromatographic similarity to the target. If the number of descriptors calculated for each database compound is large (Dragon software calculates up to 6,000 descriptors) a genetic algorithm is used to findthose descriptors that are most relevant to the prediction of retention times. Next the QSRR model is developed to provide an equation relating retention time to the relevant descriptors using an appropriate statistical tool, such as partial least squares regression. Finally, the model is then used to predict the retention time of the target compound by inserting the values of the relevant descriptors into the QSRR equation.
Who will benefit?
The application areas are unlimited. Perhaps the most obvious application lies in the pharmaceutical sector, where chromatographic separations are a lynchpin of successful drug development from early drug discovery through to final quality control of a manufactured drug product.
Consider the situation where an early drug discovery team has identified a target molecule to treat a specific disease. Drug synthesis modeling software has suggested three different synthesis routes that might be used to make the target molecule and has also suggested the range of impurities that are likely to be generated with each synthetic route. If the drug development chemists could use the QSRR approach to predict the retention times of the target molecule and the associated impurities from each synthesis route they could predict whether separation difficulties (separation of impurities from each other or from the target molecule) are likely to emerge in later stages of drug development, especially in quality control, and avoid those routes of synthesis.
Another good example lies in the area of non-targeted metabolomics. Here, metabolite screens are used to identify a target metabolite peak in that might be related to a particular disease. Mass spectroscopic analysis of the target peak might suggest multiple candidate chemical structures for the metabolite and the only way to eliminate false positives amongst the suggested candidates is to measure their retention times to see if they are eluted at the same retention time as the target metabolite peak. Using the QSRR approach the retention times of the suggested candidates can be predicted and false positives can be eliminated.
Manufacturers of chromatographic instruments and columns could also reap the benefits of retention prediction; customers often ask, “What is the best column and mobile phase for me to use for my particular separation?” QSRR would allow the in-silico screening of multiple stationary and mobile phases to come up with a logical selection of the best conditions.
It should come as no surprise then that the industrial partners sponsoring this research comprise a major pharmaceutical company (Pfizer Inc), a major scientific instrument manufacturer (Thermo Fisher Scientific) and a well-known developer of chromatography software (LCResources)! Each one of these partners has played a vital role, such as identifying scenarios for the application of QSRR modeling, the provision of databases of retention times of compounds, assistance with software, and provision of columns for testing of predictions. It has been a genuinely collaborative project.
What’s next?
We have now completed the proof-of-concept phase of the project. We have shown that the QSRR approach – when applied with suitable database clustering methods – allows the prediction of retention times with an error of under 5 percent in reversed-phase LC, HILIC and ion-exchange chromatography. We have demonstrated applications in HILIC method development, early-stage pharmaceutical product development and non-targeted metabolomics.
However, we have been somewhat limited by the size of available retention databases, typically 150 compounds. Limited size means limited compound diversity, which sometimes leaves us unable to identify a sufficiently large enough number of “similar” compounds for the training set for some target compounds. The next stage of the project will be to compile larger and more structurally diverse databases. Here, we will likely look to crowdsourcing or the establishment of a consortium of interested industrial partners (such as a group of pharmaceutical companies) who would be willing to share retention data. The larger the final database the better, provided that the retention data are reliable. With a big enough database, the sky’s the limit.
Paul Haddad is Distinguished Professor of Chemistry at the University of Tasmania, and from 2001-2013 was the Foundation Director of the Australian Centre for Research on Separation Science. He has worked in IC for more than 30 years, with a special emphasis on the development of algorithms for computer- assisted prediction of retention times in IC.