Wrangling Orthogonality in Multidimensional Chromatography
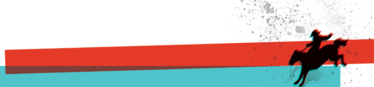
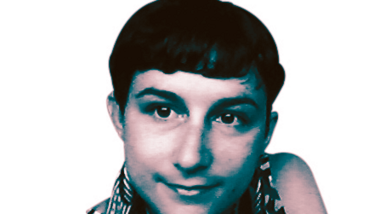
The quest for greater separation power, in terms of peak capacity, has inevitably led to the development of multidimensional chromatographic techniques. However, the increase in separation power comes with a cost: more time and/or effort is required to fully optimize multidimensional chromatographic systems. Much attention has been given to developing protocols and techniques for optimizing instrumental aspects, particularly modulation between the separation dimensions and the optimization of gradient elution profiles to increase selectivity. And though it is clearly important to improve our understanding of these aspects, it is arguably equally important that we understand and appreciate the role of orthogonality.
The concept of orthogonality refers to the combination of separation modes – or selectivities – that are independent from each other in terms of their retention mechanisms. The use of independent separation mechanisms allows different properties of the sample components to be exploited thus improving our ability to separate them. Truly orthogonal two-dimensional systems provide the potential to gain access to the theoretical maximum possible peak capacity as given by the equation from Giddings (1):
2Dnc = 1nc x 2n
In practice, a number of factors prevent us from reaching the “Holy Grail” of theoretical maximum peak capacity, and so variations on the above equation have been proposed to take such factors as dilution during modulation and difficulties in coupling truly orthogonal selectivities into account. However, such discussions are outside the scope of this article. The “take-home” message here is that to move towards the theoretical maximum, we need orthogonal selectivities.
The consequence of combining similar (or worse, identical) selectivities is that sample components will cluster along the diagonal of the separation space and thus not utilize the full peak capacity potential. Therefore, we must understand orthogonality and consider it fully during optimization. Orthogonality metrics are useful for this, particularly in industry, where method development is often guided by the need to achieve specific values for system suitability parameters. The problem lies in the choice of orthogonality metrics. I have read of numerous instances where an analyst has not fully understood the concept of orthogonality and has used the wrong metrics, leading to a biased measure of orthogonality. Even worse is when an analyst makes an incorrect claim of orthogonality – for example, stating that IMS is orthogonal to MS in IMS/MS. That is simply not true. Both IMS and MS involve a mass-based separation despite the fact that IMS also separates in terms of shape. These separation mechanisms are correlated and therefore not orthogonal, which is why peaks in an IMS/MS spectrum cluster along the diagonal.
Many orthogonality metrics exist and unfortunately there is inadequate space here to review them fully; however, the criteria I personally use to determine the best metric are as follows:
- Easy to implement
- Relates physically to the concept of orthogonality. That is, it should directly link to the concept of orthogonality; not model or describe orthogonality based on descriptors that do not make physical sense
- Freedom from user bias
- Independent from the number of components within the sample
- Should be precise
- Clustering of peaks around the upward leaning diagonal is not assumed
- The metric should be insensitive to region where there are no peaks
- Should describe the separation in question (not probe solutes).
In my opinion, there are only two orthogonality metrics that come close to satisfying these criteria completely: the bin counting methods developed by Gilar, Stoll and colleagues (2, 3) and the recently introduced asterisk approach, which I developed with Peter Schoenmakers (4). The main advantages that the asterisk approach has over the bin counting methods is that it is not necessary to know the number of sample components beforehand and it is easy to implement. Conversely, the advantage that the Gilar, Stoll bin methods have currently over the asterisk approach is that they are applicable for samples containing less than 25 components.
Whatever the multidimensional separation technique used, it is essential that we fully understand orthogonality and choose an appropriate metric to guide our method development.
The new sheriff in town by Daniel Armstrong
Wrangling orthogonality in multidimensional chromatography by Michelle Camenzuli
Reigning in multidimensional data by James Harynuk
Vacuum-uv lone star versus ms by Kevin Schug
Rodeo champs by Mark Schure
Young guns: ionic liquids for gcxgc by Jared Anderson
- J. C. Giddings, “Two-Dimensional Separations: Concept and Promise”, Anal. Chem., 56, 1258A-1270A (1984).
- M. Gilar et al., “Orthogonality of Separation in Two-Dimensional Liquid Chromatography”, Anal. Chem., 77, 6426–34 (2005).
- J. M. Davis, D. R. Stoll and P.W. Carr, “Dependence of Effective Peak Capacity in Comprehensive 2D Separations on the Distribution of Peak Capacity Between the Two Dimensions”, Anal. Chem., 80, 8122–34 (2008).
- M. Camenzuli and P. J. Schoenmakers, “A New Measure of Orthogonality for Multi-Dimensional Chromatography”, Anal. Chim. Acta, 838, 93-101 (2014
Michelle Camenzuli, van ’t Hoff Institute for Molecular Sciences, University of Amsterdam, The Netherlands.