Will AI Help – or Hinder – the Chemometrics Field?
The jury’s still out, says ACD/Labs’ Graham A. McGibbon – who points to prompt engineering and difficulties obtaining high-quality instrument data as current hurdles
| 5 min read | Interview
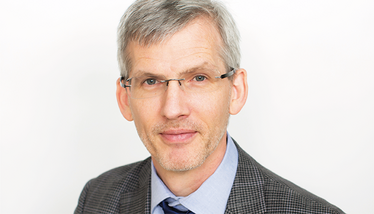
Graham A. McGibbon
What sparked your interest in chemometrics?
My education focused on chemistry, math, and computer science and it became evident in my own pharma and academic research, but also through many conversations with ACD/Labs’ customers, that analytical instrument data is vital in understanding the composition of (bio)chemical substances and materials. To obtain understanding one needs powerful software tools to deal with feature extraction and selection, as well as reduction or regression and classification.
What is your definition of chemometrics and how does it differ from AI and machine learning?
I consider chemometrics to be the application of advanced statistical analyses to sets of chemical data, particularly via linear multi-variate data modeling. Advanced statistics transcend the usual accuracy and precision calculations.
AI is typically considered a high-level general category of data modeling – large language models are one common aspect. Machine Learning is a subset of AI describing automated systems that can adjust (and ideally improve) data models based on adding new data features or classification information. Although automation can involve chemometrics linear models, ML typically leverages non-linear models such as shallow or deep neural networks to predictively classify or generatively create new entities.
What are some big problems in analytical science that we can solve with chemometrics?
One major issue facing suppliers and consumers across a large number of industries is knowing whether materials are authentic or not. We have all read about tragedies related to the nefarious adulteration of foods. Concerns about counterfeit medications and other drugs are also often in the news. Also, there is significant interest in establishing geographic or supply chain origins of materials to ensure product quality and authenticity. For example, spectra of foods or ingredients that grow in certain regions and are prepared by specific processes have commonalities from plant/animal types, environment, soil, moisture, equipment, and so on, which can allow grouping by principal components analyses or k-means clustering that discriminates them from items grown elsewhere or made differently.
What are the major hurdles to making progress in chemometrics?
Data accessibility is still a problem in some labs that lack systematic storage, query and retrieval systems. While chemometrics generally applies well to small data collections, it’s unusable if the tools and the data cannot be brought together easily enough.
Effective data management not only enables access to the relevant pieces of analytical data, but also metadata, and any interpretation results. Being able to jump easily to different types and sets of data will facilitate chemometrics data modeling for feature extraction and selection.
Can you highlight some of the key trends or chemometric tools or advancements currently being used to overcome these challenges?
Shifting or missing data are challenges for both chemometrics and ML, so tools that tackle these aspects are of increasing interest – such as the several considered by Kharbach et al. in a 2023 review of chemometrics for food. For example, extensions of parallel factor analysis and point matching are receiving attention for shifting in separations. As Kharbach et al. point out, addressing missing data is so difficult that many approaches, some global and others local, have been reported and no single one is most notable yet for addressing the issue.
What role will AI play in the future of chemometrics?
AI certainly provides a powerful approach to modeling data. For the future of chemometrics it remains to be seen if some essential ingredients will mature sufficiently: firstly, how much domain-specific tailoring will be needed for excellent generative prompt engineering; and secondly, whether sufficient sets of high-quality analytical instrument reference data can be obtained and maintained to get beyond the current state of data acquired as needed for the problems at hand. Since chemometrics is a subset of AI focusing mostly on using linear methods of statistical analyses of datasets, it may grow with or be impeded by AI growth of other methods (e.g., ML), so I don’t think we know which way it will play out yet.
Is the current hype around AI having an impact on your field?
AI hype is certainly driving interest in our field of analytical data management. The root problem for the majority of R&D organizations is the data: it is heterogeneous, typically scattered, sometimes inconsistently managed and perhaps of variable or even dubious quality and completeness. After the first wave of covid-fueled interest in better data management, there has been a second wave of realization that data need to be acquired and managed in systematic ways if they are to be extractable and structured for data science. A foundation of standardized curated data with contextual experimental metadata is essential for reliable insights from AI applications.
Looking ahead, what are you most optimistic about when it comes to chemometrics?
We are starting to see some possibilities of selecting between or combining chemometrics and ML approaches. I expect that having environments that allow either approach to be available and easily used according to the accessible data set sizes and the nature of the questions being asked will benefit not only chemometricians but all data scientists.
Graham A. McGibbon is Director, Strategic Partnerships, ACD/Labs.
Graham has a PhD in analytical chemistry. Building on an education in chemistry and mathematics, he has conducted research in academia, instrumentation, and anti-viral discovery in big pharma. He joined ACD/Labs over 17 years ago as a software product manager and after several years transitioned to the current role. Presently, he helps develop and maintain successful strategic relationships and collaborative initiatives between third-parties and ACD/Labs teams to enhance software innovation. This includes business dialog with partners and clients to help obtain insights and deliver on needs, R&D workflows, software requirements, improvements in technologies and products.
Acknowledgements also to Sanji Bhal, Director of Marketing & Communications, ACD/Labs