Targeting the Untargeted
Our capacity to generate data is unsurpassed, but how do we cope with the data deluge? It’s time to embrace data-driven discovery in biology and medicine.
The rising areas of systems, synthetic, and chemical biology offer an exciting prospect. With allied advances in molecular biology, such as rapid genome editing, the questions posed of biology have increased in their breadth. Our potential to understand the answers to those questions may lie directly in our ability to observe and translate complex biological responses as objectively as possible. But purely compartmentalized, hypothesis-driven research tends to suffer from a subjective bias towards what is being asked and how we are listening for the answers. Such targeted analysis is like a Rosetta stone that may – or may not – hold all the key characters. In contrast, big data generation and interrogation strategies promote the concept of measuring all that we can and allowing the data to drive discovery. There is, of course, a continuum from specific hypotheses to data-driven discovery.
Four elements make untargeted analyses suitable for driving new discovery in biology and medicine: (i) the increased prevalence of instrumentation and hyphenated techniques that are capable of generating high dimensional datasets, (ii) the opportunities for interdisciplinary advances in big data strategies that can be imported from fields such as astronomy, business, and systems theory, (iii) the abstraction of salient biological information from complex biological “noise,” and (iv) the iterative refinement of coarse-grained untargeted analyses to develop fine-grained understanding of specific hypotheses.
Research over the past several decades to interface distinct approaches (often with disparate operating characteristics, such as flow rates and pressures) has resulted in many contemporary studies that integrate techniques much like individual building blocks. We can now pair the most selective separation mechanism with the most sensitive detector even for complex samples. In other words, the rise of hyphenated strategies provides a means to tailor the analytical approach to the experiment at hand, rather than the other way around. Guided by lab-on-a-chip and microfluidic platforms, we can also scale the analytics appropriately to many questions asked in biology and medicine, ranging from measurements on tissue biopsies to single cells and cell cultures, to replicating human physiology in “organs-on-chip” and “human-on-chip” efforts. In all of these cases, the sample sizes are vanishingly small and yet the samples are exceedingly complex.
A variety of strategies, including chip-based genomics and mass spectrometry detection, provide data rates on the order of 104 to 105 detected hits or peaks within minutes or greater than 106 to 107 molecular features per hour. Generating data density at this rate vastly surpasses our ability to interrogate, identify, and validate each and every signal that is recorded. Indeed, the double-edged sword of untargeted analyses is that in the deliberate attempt not to miss hitherto unknown biology by measuring all that we possibly can, a tremendous amount of “noise” is generated in the measurement. In this context, noise can be considered anything that does not pertain to the question being asked and can arise from a variety of sources, including the biology itself and the superposition of biological function – how does one parse inflammatory response signals from those at the root cause of the inflammation?
Clearly, we must in order to translate the sea of data into signals that contain pertinent information – a task that is not dissimilar to contemporary research directions in areas such as astronomy or even Internet marketing. In fact, direct analogies can be drawn from the data-mining of Internet usage for advertising and commerce; the best way to make accurate, individualized purchasing recommendations is to compare enormous datasets of page views, searches and purchasing patterns for large numbers of customers and to recommend the last action of one individual to the individual with the most closely related pattern. Increasingly, these tasks are performed by strategies that use the self-organization of data to sort salient features from the noisy data. Many of these strategies are beginning to find application in biology and medical research – a trend that is likely to continue in the foreseeable future.
One of the well-acknowledged challenges of big data strategies is that while self-organization of data can reveal otherwise unknown trends and relationships, it is tantamount to observing correlation rather than implying causation. Therefore, this coarse-grained view of the massive dataset should be used to focus on a smaller subset of signals that likely contain the answers that are sought. Iterative interrogation, identification, and validation of those subset signals is then critical to gain insight into the system and to refine hypotheses.
Many exciting avenues are being opened up by data-driven discovery. And we are only just at the beginning; new paradigms for parsing high-dimensional data in near-real time may be necessary as studies increasingly weave spatial and dynamics information from complex biological or ecological interactions into the broad tapestry of questions we are now wanting to ask.
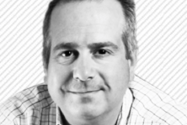
“Throughout my childhood I was curious about the nature of things, but more in areas like economics and political science.When I was in my 20s,a persuasive series of teachers and mentors lit my passion for chemistry.”McLean began his research career in plasma spectrochemistry and later moved into biological mass spectrometry where he and his group colleagues perform research in instrumentation construction for application areas in biology and medicine. “There are few more exciting things in life than working with enthusiastic student colleagues and aggressively asking questions that can change how we think about the world around us.”